Autism is a tough nut to crack.
Part of the reason is complexity: The disorder manifests in a bewildering array of symptoms — everything from basic perceptual disturbances to high-level cognitive and social deficits. Because of this heterogeneity, the hunt for so-called autism genes and molecules in autistic patients has been rather unfruitful.
Yet to some scientists, the disorder’s complexity is actually a clue in disguise.
Autism is systems-wide, noted Dr. Ari Rosenberg, a computational neuroscientist at the Baylor College of Medicine in a recent publication in PNAS. That’s a valuable hint, says Rosenberg. Instead of narrowly impacting a particular function, autism must change some fundamental neural code — a kind of canonical algorithm that’s embedded in multiple computations across the brain.
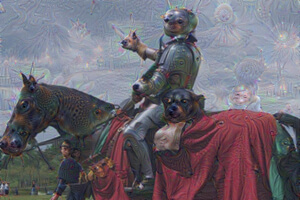
To debug the corrupt neural base code, Rosenberg and colleagues turned to a highly unconventional tool in psychiatry: artificial neural networks (ANNs).
From previous studies, the team already knew that people with autism behaved differently in several psychophysiological visual tasks. It’s a clue that early visual processing in autistic people is somehow out of whack. Coincidentally, the primary visual cortex (V1) also happens to be a prime area for modeling with ANNs, perhaps in part due to its popularity with the machine learning crowd.
All they had to do, Rosenberg reasoned, is build a simple, single-layer artificial V1, feed data from previous psychology studies into it, then tweak parameters in the model’s algorithm and see if they could reproduce “autistic behavior.”
But what exactly is the buggy brain computation? One property — divisive normalization — stood out as a candidate. Normalization is simple but crucial: it allows activated neurons to dampen the signal of other neurons in its network. In essence, it boosts the signal-to-noise ratio and guards against runaway excitation in the brain.
Reducing autism to a matter of signal “gain” may seem over-simplistic, but it’s a good place to start. For one, there’s ample evidence from brain imaging studies that at least some regions in the autistic brain are hyperactive compared to a neurotypical one. What’s more, in artificial intelligence, in order for biologically realistic, hierarchical artificial neural networks to perform complex object recognition tasks, normalization-like procedures are required at every level. On an operational level, normalization could also be tweaked by changing a single parameter, making the model computationally tractable. It’s a great test case.
Rosenberg began his experiment by presenting the ANN with dynamic gratings of light and dark lines and asked it to report which way the grating moved.
In general, autistic people outperform their neurotypical counterparts in this task, especially when the contrast between dark and light lines is high. When the team turned down normalization in their model to mimic the autistic brain, they observed exactly the same trend — that is, the “autistic” ANN was better at picking out the movement than the “normal” network.
The team saw similar results with a “tunnel vision” test: When they decreased normalization, the ANN — just like autistic people — paid less attention to visual stimuli that occurred far from the point of fixation than a typical visual cortex.
Finally, the team also tested top-down control of visual processing.
They exploited a known trait in autism: People with autism rely far less on past experiences (known as “Baysian priors” in AI) when interpreting current sensory information. To see if they could recapitulate this effect, the team fed information to their ANN about what to expect from a visual stimuli.
Like human behavioral results, the “autistic” ANN — with normalization turned down — benefited far less from the prior than its “normal” counterpart.
In all three tests, ANNs with decreased normalization seem to function like humans beings with autism. This really shows how digital modeling can help us better understand experimental data, says Dr. Jeroen van Boxtel, a cognitive neuroscientist at Monash University who was not involved in the study.
But it’s critical to note the model is only a simplified metaphor of what really goes on in the enormously complex jungle that is the autistic brain. Buggy normalization and Bayesian inference certainly seem like enticing culprits underlying computational deficits in autism, but how they’re implemented biologically in our fatty, squishy brains remains to be seen.
As of now, the similarities between the ANN and autistic brains could be correlation and not much else. It falls on neurobiologists to decipher the molecular pathways responsible for the faulty algorithms (if they exist) the old way — by tweaking one protein variable at a time.
Yet van Boxtel welcomes the crossover between psychiatry, computational neuroscience and AI. “Modeling — like any type of coding — forces one to clearly state one’s assumptions in a highly logical manner,” van Boxtel said to Singularity Hub in an email. But most importantly, it generates highly specific mechanistic hypotheses that allow future scientists to make predictions and test them out. Because an ANN model only has a few components, it’s easy to figure out which ones are important for a certain effect and rule out confounders.
“The model the authors propose is based on well-supported, previously-published models, and stands a good chance of providing an explanation for future work as well,” says van Boxtel.
Dr. Matteo Carandini, a computational neuroscientist at the University College London who studies cortical information processing, agrees. The increasing sophistication of brain mapping tools is propelling efforts to reconstruct a whole human brain in unprecedented detail. Just last week, a team published the first complete 3D map of a piece of tissue in the mouse’s neocortex, and the various technologies involved will no doubt tremendously speed up over the next decade.
Other efforts are mapping wide-reaching functional networks — called “connectomes” — by gamifying the process and crowdsourcing data from citizen scientists.
Carandini is excited: This explosion of structural and functional neural network data will rapidly lead to better models. With President Obama’s BRAIN Initiative and the European Union’s Human Brain Project actively promoting in silico brain simulations, the time is ripe for computational approaches to become part of the “standard package” in neuroscience research.
There’s no doubt that both big data brain initiatives have received quite a lot of criticism due to their moonshot nature and unclear goals. And so far, concrete examples of fruitful brain simulations are few and far between. Yet van Boxtel and Carandini both believe that computational models — however simple — have the power to guide psychiatry.
“A computational approach to disease?” says Carandini. “I think it is long overdue.”
Image Credit: Shutterstock.com