“I consider that a man’s brain originally is like a little empty attic, and you have to stock it with such furniture as you choose… It is a mistake to think that that little room has elastic walls and can distend to any extent. Depend upon it there comes a time when for every addition of knowledge you forget something that you knew before. It is of the highest importance, therefore, not to have useless facts elbowing out the useful ones.” — Sherlock Holmes in Sir Arthur Conan Doyle’s “A Study In Scarlett”
According to Dr. Terry Sejnowski, a pioneer in computational neuroscience at the Salk Institute, your brain can hold petabytes of data — an order of magnitude similar to the entire internet.
It’s a bombshell of a claim, one that’s sexy, easy to comprehend, and solidly rooted in the “brain as computer” analogy. Yet according to Dr. Paul Reber, a neuroscientist at Northwestern University, the claim overshadows the remarkable science behind the study.
Some media reported that this new estimate of the brain’s storage capacity is ten times more than previously thought, but it’s actually remarkably similar to what we’ve guessed before. I also think it’s off, but that’s ok, laughed Reber.
In this case, “how much” is far less fascinating than “how.” How does the brain achieve that impressive storage power? How do we fill that storage? How — if at all — can unraveling the brain’s storage secrets guide us towards more efficient computer algorithms?
The answers, surprisingly, are etched into the brain’s nanoscale organization.
Size = strength
According to modern neuroscience, our dearest memories are stored within the connections between networks of neurons.
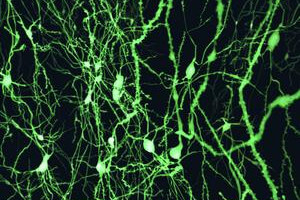
These connections are not abstract weights, such as those in an artificial neural network. Instead, they are physical in nature. Called synapses, they rely on many proteins working together at small protrusions on the neurons’ dendrites called spines. If neurons are single processing units, dendrites are cables that extend out from the unit body, and spines are points-of-contact where signals transform and transmit.
A fundamental idea in neuroscience is “fire together, wire together.” If a stimuli — say, the taste of coffee — causes a group neurons to activate together, the connections between them become stronger.
In an artificial neural network, “stronger” means that synaptic weights become numerically larger; in a biological one, the synapses physically grow larger. The spines — mushroom-shaped protrusions that synapses sit on — also bloom in size.
We know synapse size correlates with strength and storage capacity; we can even watch them grow under fancy microscopes, explained Dr. Tom Bartol, lead researcher of the new memory capacity study published last week in eLife.
The question is: if synapse strength is a proxy for information storage, how accurately can we retrieve that information by measuring the size of the synapse?
Depending on how much information is stored, our guess is that the physical size of the synapse is different; that is, the sizes are in variable states, explained Sejnowski to Singularity Hub.
But synapses were previously thought to only come in three flavors: small, medium and large. No one knew if sizes existed in more discreet states or laid on a continuum.
No one’s ever done geometrically accurate reconstructions of synapses before, and that’s what we did, said Sejnowski.
Complex and precise
The team set out to reconstruct every neuron and synapse from a chunk of the hippocampus — the brain region involved in learning and memory — at roughly the scale of a red blood cell.
Funny thing, we were using those reconstructions to do computer simulations of neurons, not to measure the brain’s storage capacity, laughed Sejnowski.
But when Bartol pored over the images, he noticed something interesting. Although most neurons paired up one-to-one, in some cases, one neuron would form two different synapses with another, suggesting that it was sending duplicate messages.
Because those pairs of synapses have the same activation history, we thought they’d be roughly similar in size, said Bartol. Actually, in a perfect environment they’d be exactly the same, he explained.
But the brain’s messy. Random events, such as faulty proteins, can easily lead to noise and degrade the precision of synaptic strength.
Yet nature threw Bartol a giant curveball.
“We were amazed to find that the difference in the sizes of the pairs of synapses were very small, on average, only about eight percent different in size. No one thought it would be such a small difference,” said Bartol.
In other words, information storage in the brain is shockingly precise.
The team then carefully examined the range of synapse sizes in their reconstruction, which differed by a factor of 60 between the smallest and the largest. Using signal detection theory, Bartol estimated that synapses could exist in as many as 26 distinct states.
By just looking at the sizes of synapse, the brain (and us!) can guess how much information is stored in the connection and retrieve it precisely.
“The implications of what we found are far-reaching,” said Sejnowski. “Hidden under the apparent chaos and messiness of the brain is an underlying precision to the size and shapes of synapses that was hidden from us.”
Averages over singles
The team went on to guesstimate the brain’s storage capacity with a few major assumptions, including that synapses work in the same way in other brain regions as in the hippocampus. This led to their petabyte estimate.
It’s a fun result and one that’s easy to understand when we compare the brain with computers, said Reber. There’s one practical difference: the precise number of computer storage matters; our brains, not so much.
For all intents and purposes, we might as well consider memory storage infinite for our lifetime, said Reber.
To Sejnowski, the difference between how the brain achieves its precision compared to a computer is far more intellectually rewarding.
Synapses are notoriously unreliable, explained Sejnowski. Say a random neuron fires; only 10-20% of the time does it trigger the next one to also fire. Plug those success rates into our computer systems and you cripple them.
If we only look at a single point in time, the brain is incredibly messy. So how does it achieve its remarkable precision?
The answer seems to be averaging over time. Using their new data and statistical models, the team found that it took roughly 1 to 2 minutes and thousands of individual signals for a pair of synapses to achieve the 8% difference they had previously measured.
In other words, it’s the averaged out value of synaptic strength that is significant, not each individual transmission. This reduces the effect of single transmission mistakes — think one randomly flipped bit in software — and makes the brain much more robust than traditional computers.
It also slashes the amount of energy required for computation.
“It should be possible to build deep learning networks that use probabilistic transmission like the brain to achieve good performance for a fraction of the computational cost,” said Sejnowski.
As a computational neuroscientist, Sejnowski doesn’t shy away from the brain-computer analogy.
Obviously it’s not perfect, but it helps us understand how the brain processes information without diving down into the molecular level, which is important but also a mess of a molecular soup. Computational terms are much easier to understand and explain.
But the best use for the analogy may be that we can use findings from neuroscience — the only natural intelligence system that we’re aware of — to guide the development of artificial intelligent systems.
It’s god’s gift to science, laughed Sejnowski.
Image Credit: Shutterstock.com