What Anthropic Researchers Found After Reading Claude’s ‘Mind’ Surprised Them
As AI's power grows, charting its inner world is becoming more crucial.

Image Credit
Despite popular analogies to thinking and reasoning, we have a very limited understanding of what goes on in an AI’s “mind.” New research from Anthropic helps pull the veil back a little further.
Tracing how large language models generate seemingly intelligent behavior could help us build even more powerful systems—but it could also be crucial for understanding how to control and direct those systems as they approach and even surpass our capabilities.
This is challenging. Older computer programs were hand-coded using logical rules. But neural networks learn skills on their own, and the way they represent what they’ve learned is notoriously difficult to parse, leading people to refer to the models as “black boxes.”
Progress is being made though, and Anthropic is leading the charge.
Last year, the company showed that it could link activity within a large language model to both concrete and abstract concepts. In a pair of new papers, it’s demonstrated that it can now trace how the models link these concepts together to drive decision-making and has used this technique to analyze how the model behaves on certain key tasks.
“These findings aren’t just scientifically interesting—they represent significant progress towards our goal of understanding AI systems and making sure they’re reliable,” the researchers write in a blog post outlining the results.
The Anthropic team carried out their research on the company’s Claude 3.5 Haiku model, its smallest offering. In the first paper, they trained a “replacement model” that mimics the way Haiku works but replaces internal features with ones that are more easily interpretable.
The team then fed this replacement model various prompts and traced how it linked concepts into the “circuits” that determined the model’s response. To do this, they measured how various features in the model influenced each other as it worked through a problem. This allowed them to detect intermediate “thinking” steps and how the model combined concepts into a final output.
In a second paper, the researchers used this approach to interrogate how the same model behaved when faced with a variety of tasks, including multi-step reasoning, producing poetry, carrying out medical diagnoses, and doing math. What they found was both surprising and illuminating.
Be Part of the Future
Sign up to receive top stories about groundbreaking technologies and visionary thinkers from SingularityHub.
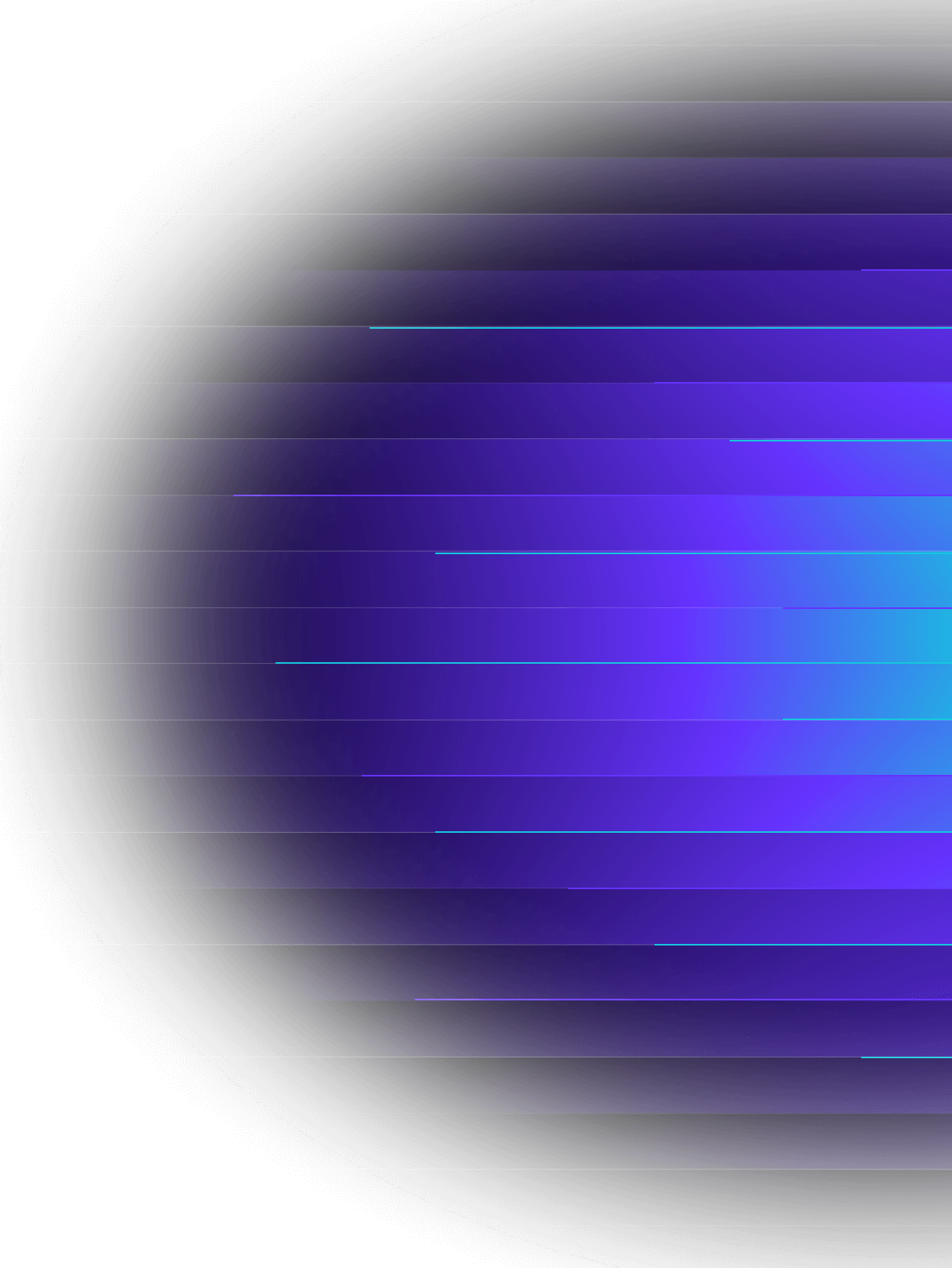
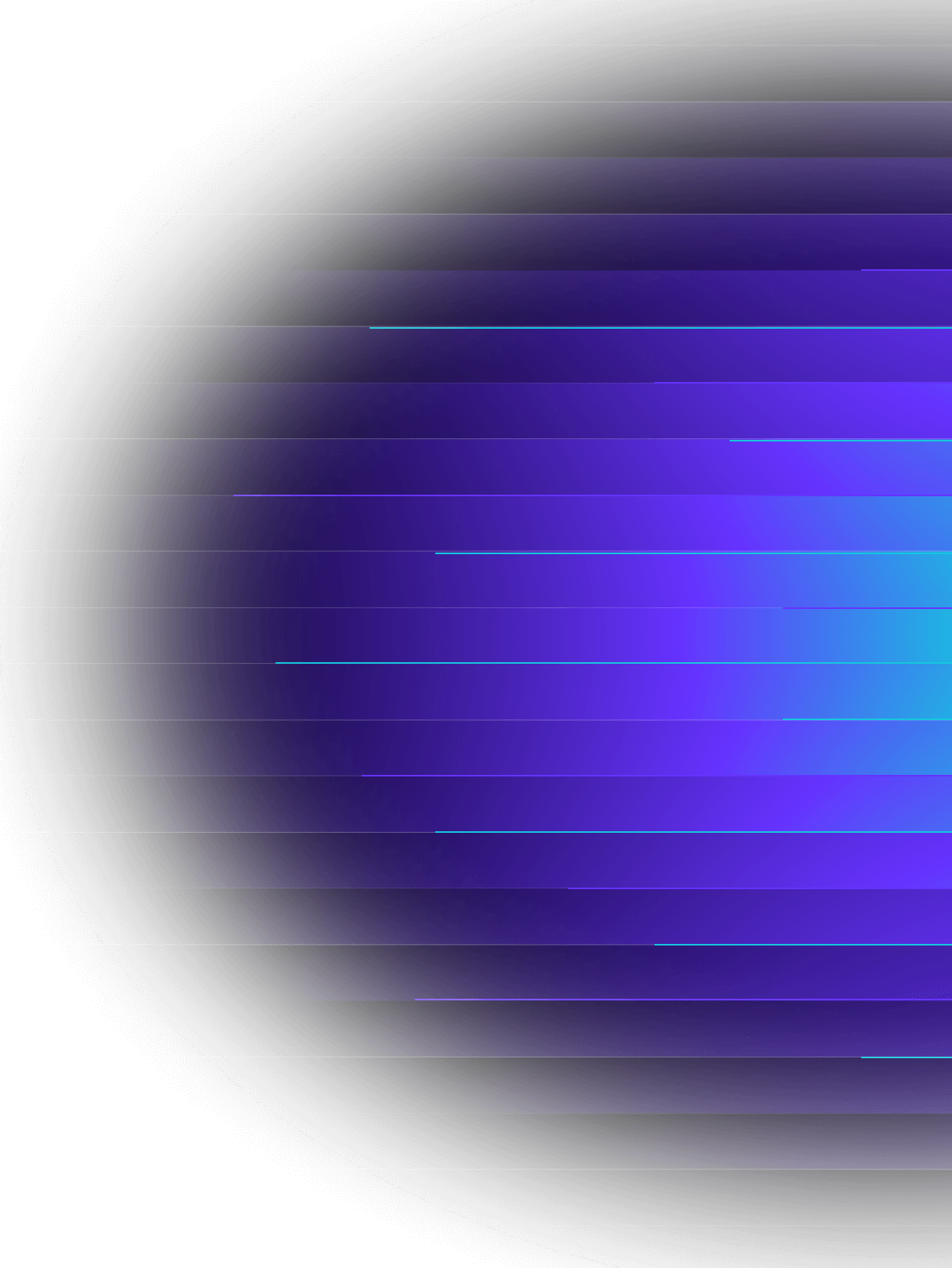
Most large language models can reply in multiple languages, but the researchers wanted to know what language the model uses “in its head.” They discovered that, in fact, the model has language-independent features for various concepts and sometimes links these together first before selecting a language to use.
Another question the researchers wanted to probe was the common conception that large language models work by simply predicting what the next word in a sentence should be. However, when the team prompted their model to generate the next line in a poem, they found the model actually chose a rhyming word for the end of the line first and worked backwards from there. This suggests these models do conduct a kind of longer-term planning, the researchers say.
The team also investigated another little understood behavior in large language models called “unfaithful reasoning.” There is evidence that when asked to explain how they reach a decision, models will sometimes provide plausible explanations that don't match the steps they took.
To explore this, the researchers asked the model to add two numbers together and explain how it reached its conclusions. They found the model used an unusual approach of combining approximate values and then working out what number the result must end in to refine its answer.
However, when asked to explain how it came up with the result, it claimed to have used a completely different approach—the kind you would learn in math class and is readily available online. The researchers say this suggests the process by which the model learns to do things is separate from the process used to provide explanations and could have implications for efforts to ensure machines are trustworthy and behave the way we want them to.
The researchers caveat their work by pointing out that the method only captures a fuzzy and incomplete picture of what’s going on under the hood, and it can take hours of human effort to trace the circuit for a single prompt. But these kinds of capabilities will become increasingly important as systems like Claude become integrated into all walks of life.
What we’re reading