From AlphaGo’s historic victory against world champion Lee Sedol to DeepStack’s sweeping win against professional poker players, artificial intelligence is clearly on a roll.
Part of the momentum comes from breakthroughs in artificial neural networks, which loosely mimic the multi-layer structure of the human brain. But that’s where the similarity ends. While the brain can hum along on energy only enough to power a light bulb, AlphaGo’s neural network runs on a whopping 1,920 CPUs and 280 GPUs, with a total power consumption of roughly one million watts—50,000 times more than its biological counterpart.
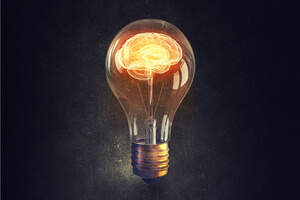
Extrapolate those numbers, and it’s easy to see that artificial neural networks have a serious problem—even if scientists design powerfully intelligent machines, they may demand too much energy to be practical for everyday use.
Hardware structure is partly to blame. Our computers, with their separate processor and memory units, are simply not wired appropriately to support the type of massively parallel, energy-efficient computing that the brain elegantly performs.
Recently, a team from Stanford University and Sandia National Laboratories took a different approach to brain-like computing systems.
Rather than simulating a neural network with software, they made a device that behaves like the brain’s synapses—the connection between neurons that processes and stores information—and completely overhauled our traditional idea of computing hardware.
The artificial synapse, dubbed the “electrochemical neuromorphic organic device (ENODe),” may one day be used to create chips that perform brain-like computations with minimal energy requirements.
Made of flexible, organic material compatible with the brain, it may even lead to better brain-computer interfaces, paving the way for a cyborg future. The team published their findings in Nature Materials.
“It’s an entirely new family of devices because this type of architecture has not been shown before. For many key metrics, it also performs better than anything that’s been done before with inorganics,” says study lead author Dr. Alberto Salleo, a material engineer at Stanford.
The biological synapse
The brain’s computational architecture is fundamentally different than a classical computer. Rather than having separate processing and storage units, the brain uses synapses to perform both functions. Right off the bat, this arrangement is better: it saves the energy required to shuttle data back and forth from the processor to the memory module.
The synapse is a structure where the projections of two neurons meet. It looks a bit like a battery cell, with two membranes and a gap between. As the brain learns, electrical currents hop down one neuronal branch until they reach a synapse. There, they mix together with all the pulses coming from other branches and sum up into a single signal.
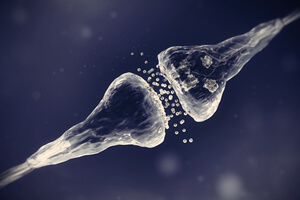
When sufficiently strong, the electricity triggers the neuron to release chemicals that drift towards a neighboring neuron’s synapse and, in turn, causes the neuron to fire.
Here’s the crucial bit: every time this happens, the synapse is modified slightly into a different state, in that it subsequently requires less (or more) energy to activate the downstream neuron. In fact, neuroscientists believe that different conductive states are how synapses store information.
The artificial synapse
The new device, ENODe, heavily borrows from nature’s design.
Like a biological synapse, the ENODe consists of two thin films made of flexible organic materials, separated by a thin gap containing an electrolyte that allows protons to pass through. The entire device is controlled by a master switch: when open, the device is in “read-only” mode; when closed, the device is “writable” and ready to store information.
To input data, researchers zapped the top layer of film with a small voltage, causing it to release an electron. To neutralize its charge, the film then “steals” a hydrogen ion from its bottom neighboring film. This redox reaction changes the device’s oxidation level, which in turn alters its conductivity.
Just like biological synapses, the stronger or longer the initial electrical pulse, the more hydrogen ions gets shuffled around, which corresponds to larger conductivity. The scalability was welcomingly linear: with training, the researchers were able to predict within one percent of uncertainty the voltage needed to get to a particular state.
In all, the team programmed 500 distinct conductive states, every single one available for computation—a cornucopia compared to the two-state (0 and 1) common computer, and perfect for supporting neuron-based computational models like artificial neural networks.
The master switch design also helped solve a pesky problem that’s haunted previous generations of brain-like chips: the voltage-time dilemma, which states that you can’t simultaneously get both low-energy switching between states and long stability in a state.
This is because if ions only need a bit of voltage to move during switching (low energy), they can also easily diffuse away after the switch, which means the chips can change randomly, explains Dr. J. Joshua Yang and Dr. Qiangfei Xia of the University of Massachusetts, who wrote an opinion piece about the study but was not directly involved.
The ENODe circumvents the problem with its “read-only” mode. Here, the master switch flips open, cutting off any external current to the device and preventing proton changes in the layers.
“A miniature version of the device could cut energy consumption by a factor of several million—well under the energy consumption of a biological synapse.”
By decoupling the mechanism that maintains the state of the device from the one that governs switching, the team was able to use a switching voltage of roughly 0.5 millivolts to get to an adjacent state. For comparison, this is about one-tenth the energy needed for a state-of-the-art computer to move data from the processor to the memory unit.
Once locked into a state, the device could maintain it for 25 hours with 0.04 percent variation—a “striking feature” that puts ENODe well above other similar technologies in terms of reliability.
“Just like a battery, once you charge it stays charged” without needing additional energy input, explains study author Dr. A Alec Talin.
ENODe’s energy requirement, though exceedingly low compared to current devices, is still thousands of times higher than the estimates for a single synapse. The team is working hard to miniaturize the device, which could drastically cut down energy consumption by a factor of several million—well under the energy consumption of a biological synapse.
Neuromorphic circuits
To show that ENODes actually mimics a synapse, the team brought their design to life using biocompatible plastic and put it through a series of tests.
First, they integrated the ENODe into an electrical circuit and demonstrated its ability to learn a textbook experiment: Pavlovian conditioning, where one stimulus is gradually associated with another after repeated exposure—like linking the sound of a bell to an involuntary mouth-watering response.
Next, the team implemented a three-layer network and trained it to identify hand-written digits—a type of benchmarking task that researchers often run artificial neural networks through to test their performances.
Because building a physical neural network is technologically challenging, for this test the team used the model of their neuron to simulate one instead.
The ENODe-based neural network managed an accuracy between 93 to 97 percent, far higher than that achieved by previous brain-like chips, reported the authors.
Computational prowess aside, the ENODe is also particularly suited to synapse with the brain. The device is made of organic material that, while not present in brain tissue, is biocompatible and frequently used as a scaffold to grow cells on. The material is also flexible, bendy enough to hug irregular surfaces and may allow researchers to pack multiple ENODes into a tiny volume at high density.
Then there’s the device itself, with its 500 conductance states, that “naturally interfaces with the analog world, with no need for the traditional power-hungry and time consuming analog-to-digital converters,” remarks Yang and Xie.
“[This] opens up a possibility of interfacing live biological cells [with circuits] that can do computing via artificial synapses,” says Talin. “We think that could have huge implications in the future for creating much better brain-machine interfaces.”
Image Credit: Shutterstock