The AlphaGo AI that grabbed headlines last year after beating a master of the board game Go has just been trounced 100-0 by an updated version. And unlike its predecessor, the new system taught itself from first principles paving the way for AI that can think for itself.
When chess fell to AI in the 1990s, computer scientists looking for a new challenge turned to the millennia-old Chinese game Go, which despite its simpler rules has many more possible moves and often requires players to rely on instinct.
It was predicted it would be decades before an AI could beat a human master, but last year a program called AlphaGo developed by Google’s DeepMind subsidiary beat 18-time world champion Lee Sedol 4–1 in a series of matches in South Korea.
It was a watershed moment for AI research that showcased the power of the “reinforcement learning” approach championed by DeepMind. Not only did the system win, it also played some surprising yet highly effective moves that went against centuries of accumulated wisdom about how the game works.
Now, just a year later, DeepMind has unveiled a new version of the program called AlphaGo Zero in a paper in Nature that outperforms the version that beat Sedol on every metric. In just three days and 4.9 million training games, it reached the same level that took its predecessor several months and 30 million training games to achieve. It also did this on just four of Google’s tensor processing units—specialized chips for training neural networks—compared to 48 for AlphaGo.
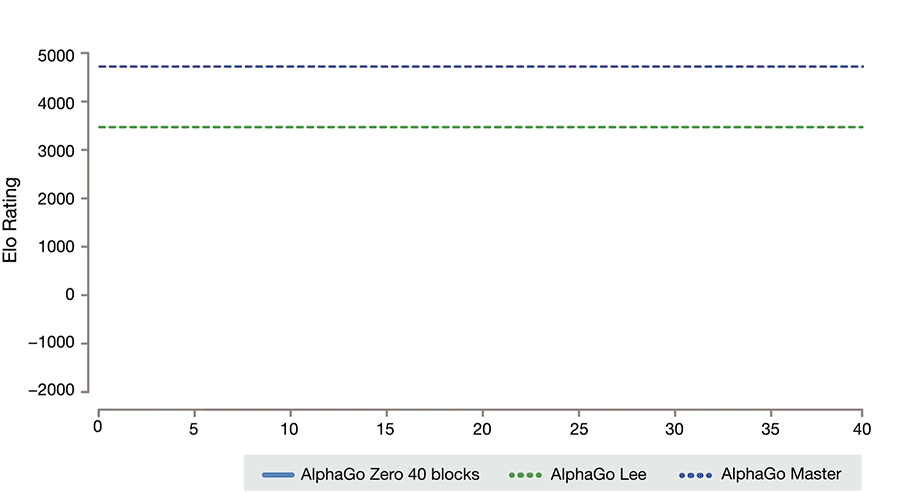
The most striking departure from the previous system is the simplicity of the inputs. AlphaGo learned the basics of Go by analyzing thousands of games between human players, before honing its skills by playing itself millions of times. In contrast, AlphaGo Zero started with nothing more than the rules of the game and learned entirely from playing games against itself starting with completely random moves.
The system’s design is not radically different from its predecessor or the later AlphaGo Master version that defeated a host of human experts, including world number one Ke Jie, which AlphaGo Zero surpassed after 40 days of training. Essentially it is a streamlining of the previous approach, enabled by a simplified architecture and more powerful algorithms.
AlphaGo features two separate neural networks. The first was trained to predict the probable best move first using the human data and then by playing itself, while the second network was trained to predict the winner of these self-play games. When it came to actually playing a game, these networks were combined with a search algorithm to drill down on the best move given the state of the game.
The first network would select the best possible moves and then the system would use a combination of the value network and “rollouts”—a series of quick simulated games to test out possible moves—to decide on a play.
The new system combines the two neural networks into a single one with many more layers of artificial neurons, which can be trained more efficiently. It also uses a much simpler search algorithm and does away with rollouts, instead relying on the higher-quality neural network to make predictions. Speaking to Nature, lead researcher David Silver likened this to asking an expert to make a prediction rather than relying on hundreds of average players to test moves out.
“It not only independently discovered known moves that have taken millennia for humans to develop, it created entirely new ones that are now redefining how Go is played.”
The fact that the researchers managed to dramatically increase performance while simplifying the system is particularly impressive, considering that many recent advances in machine learning have come from throwing more data or processors at problems. “It shows it’s the novel algorithms that count, not the computing power or the data,” Silver told the BBC.
There are the usual caveats that come with breakthroughs in AI, and in particular, reinforcement learning. The program had to play itself millions of times before it became a world-beater, many more games than a human Go player would require to reach expert level. Its achievements are also constrained to the highly ordered world of Go, which is a far cry from the messy and uncertain problems AI will eventually be asked to solve in real life.
Nevertheless, a computer that can play millions of games in a matter of days still learns immeasurably faster than a human, so this shouldn’t be seen as a major limitation. And while the transition is likely to be slow and faltering, researchers at DeepMind are already working on applying similar techniques to those at the heart of AlphaGo Zero to practical applications. In a blog post, DeepMind said the approach could hold promise in other structured problems like protein folding, reducing energy consumption, or material design.
But most importantly, the advance is the most potent demonstration so far that AI could go beyond human intelligence. In their paper, the researchers describe how when they tried training AlphaGo Zero on human games, it learned faster, but actually did worse in the long run. Left to its own devices, it not only independently discovered known moves that have taken millennia for humans to develop, it created entirely new ones that are now redefining how Go is played.
“We’ve actually removed the constraints of human knowledge and it’s able, therefore, to create knowledge itself from first principles, from a blank slate,” Silver told the BBC.
Image Credit: DeepMind