How AI Can Tap into the Collective Mind to Transform Healthcare

In 2013, IBM sold the University of Texas’ MD Anderson Cancer Center on an audacious idea: that a single AI-powered platform, IBM Watson, could lend a digital hand to battle one of mankind’s most abominable diseases—cancer.
In less than four years, the trailblazing moonshot fell apart. Yet even as Watson stumbled, it provided valuable insight—and a powerful peek ahead—at how machine learning technologies could one day supplement and revolutionize almost everything doctors do.
The question isn’t if AI is coming to medicine. It’s how. At its best, machine learning can tap into the collective experience of nearly all clinicians, providing a single doctor with the experience of millions of similar cases to make informed decisions. At its worst, AI could promote unsafe practices, amplify societal bias, overpromise on deliveries, and lose the trust of physicians and patients alike.
Earlier this month, Google’s Dr. Alvin Rajkomar and Dr. Jeffrey Dean, together with Dr. Isaac Kohane from Harvard Medical School, penned a blueprint in the New England Journal of Medicine that outlines the promises and pitfalls of machine learning in medical practice.
AI is not just a new tool, like a gadget or drug, they argued. Rather, it’s a fundamental technology to expand human cognitive capabilities, with the potential to overhaul almost every step of medical care for the better. Rather than replacing doctors, machine learning—if implemented cautiously—will augment the patient-doctor relationship by providing additional insight, they said.
Here’s the way forward.
Diagnostics to Treatment
When it comes to AI and medicine, diagnostics gets the most press.
AI-based diagnostic tools, even in their infancy, are regularly outsmarting expert radiologists and pathologists on spotting potentially lethal lesions on mammograms and diagnosing skin cancer and retinal diseases (now FDA approved!). Some AI models can even parse out psychiatric symptoms or learn to make recommendations for referrals.
These boosts in computer diagnostic prowess are thanks to recent advances in machine vision and transfer learning. Although AI radiologists normally require large annotated datasets to “learn,” transfer learning allows a previously trained AI to rapidly pick up another similar skill. For example, an algorithm trained on tens of millions of everyday objects from the standard repository ImageNet can be re-trained on 100,000 retinal images—a relatively small number for machine learning—to diagnose two common causes of vision loss.
What’s more, machine learning is well suited to analyze data collected during routine care to identify likely conditions that may pop up in the future—à la Minority Report for health. These systems could aid prevention measures, nip health issues in the bud, and decrease medical costs. When given longitudinal data of patient health in sufficient amounts and quality, AI can already build prognostic models far more accurate than any single practitioner using raw data from medical imaging.
The problem? Doctors—a relatively old-school bunch—will have to be trained on collecting the necessary information to feed into AI predictive engines, the authors said. The models would need to be carefully dissected to make sure they’re not reflecting billing incentives (more tests!) or under-suggest conditions that normally don’t manifest symptoms.
The next step in care—treatment—is much more difficult for machines. An AI model fed with treatment data may only reflect the prescription habits of physicians rather than ideal practices. A more helpful system would have to learn from carefully-curated data to estimate the impact of a certain type of treatment for a given person.
As you can imagine, that’s tough. Several recent tries found that it’s really challenging to obtain expert data, update the AI, or tailor them to local practices. For now, using AI for treatment recommendations remains a future frontier, the authors concluded.
Healthcare Overhaul
Diagnosis is just the tip of the iceberg.
Perhaps more immediately obvious is the impact of AI on streamlining doctor workflow. General AI abilities such as intelligent search engines can help fish out necessary patient data, and other technologies such as predictive typing or voice dictation—which doctors already use in their everyday practice—can ease the tedious process of capturing healthcare data.
We shouldn’t downplay that particular impact, the authors stressed. Doctors are drowning in paperwork, which hogs their precious time with patients. Educating the current physician workforce on AI technologies that increase efficiency and improve workflow could cut burnout rate. What’s more, the data can in turn feed back to train machine learning models to further optimize care for patients in a virtuous circle.
AI also holds the key to expanding healthcare beyond physical clinics. Future apps could allow patients to take a photo of a skin rash, for example, and obtain an online diagnosis without rushing to urgent care. Automated triage could efficiently shuttle patients to the appropriate form and physician of care. And—perhaps the largest hope in AI-assisted healthcare—after “seeing” billions of patient encounters, machine learning could equip doctors with the ability to make better decisions.
However, that particular scenario is only hand-waving without data to back it up. The key now, the authors said, is to develop formal methods to test these ideas without harming either physicians or patients in the process.
Be Part of the Future
Sign up to receive top stories about groundbreaking technologies and visionary thinkers from SingularityHub.
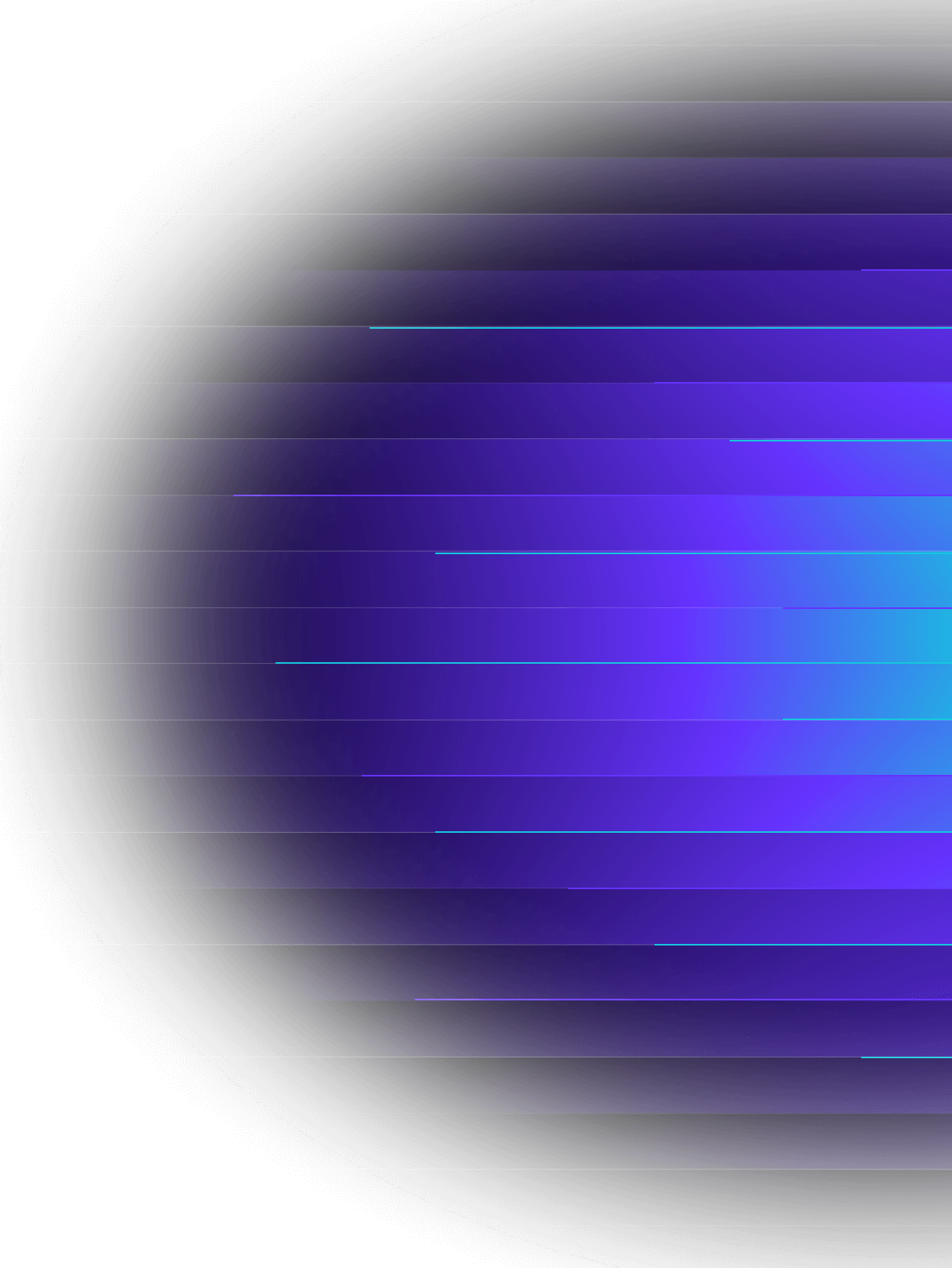
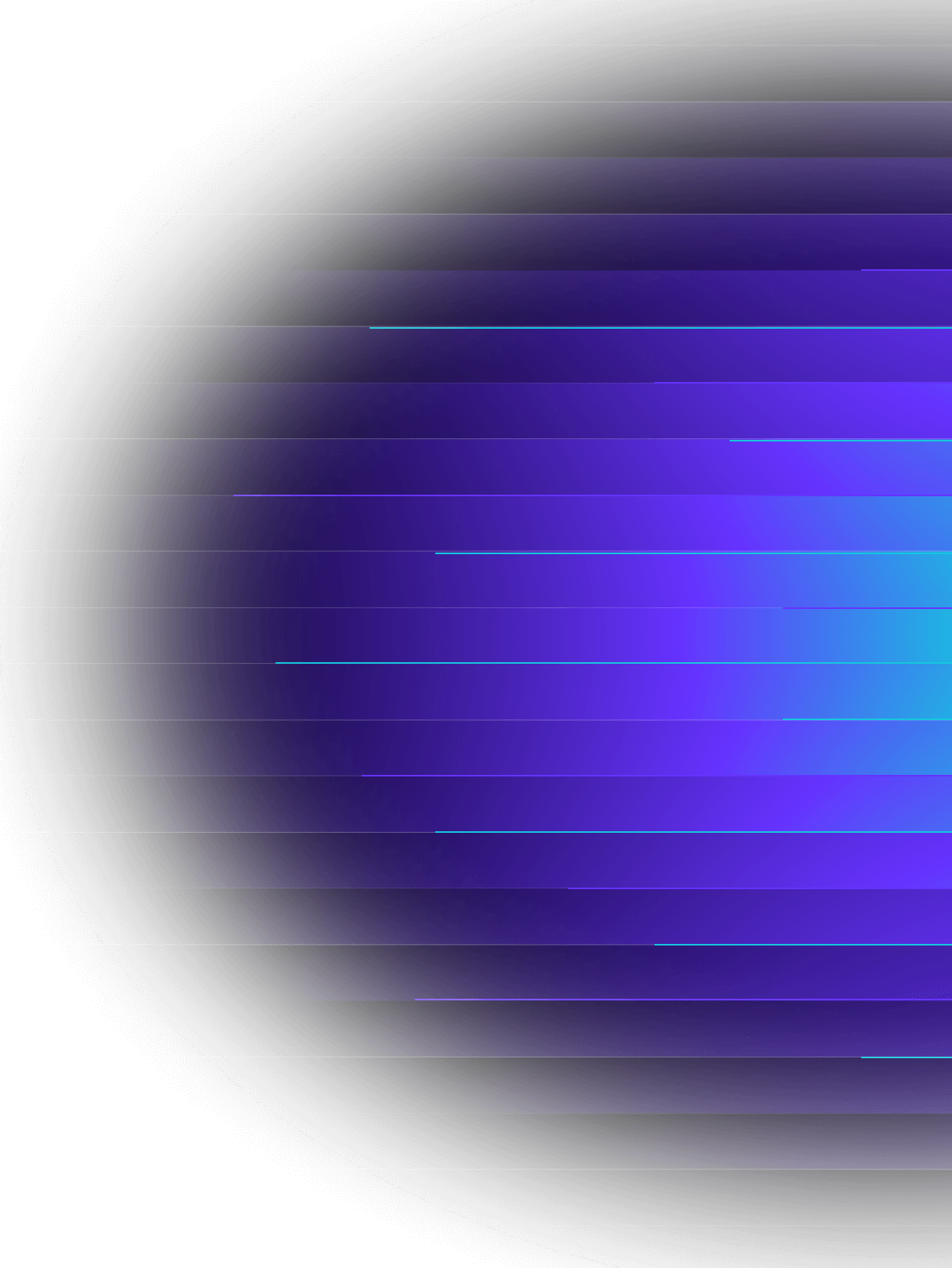
The Stumbling Blocks
As IBM Watson’s hiccup illustrates, both AI and the medical community face multiple challenges as they learn to collaborate. The Theranos fiasco further paints a painfully clear picture: when handling patient health, the Silicon Valley dogma “move fast and break things” is both reckless and exceedingly dangerous.
Medicine particularly highlights the limits of machine learning. Without assembling a representative yet diverse dataset of a disease, for example, AI models can either be wrong or biased—or both. This is partly how IBM Watson crashed: curating a sufficiently large annotated dataset to uncover yet-unknown medical findings is exceedingly difficult, if not impossible.
Yet the authors argue that it’s not a permanent roadblock. AI models are increasingly able to handle noisy, unreliable, or variable datasets, so long as the amount of data is sufficiently large. Although not perfect, these models can then be further refined with a smaller annotated set, which allows researchers—and clinicians—to identify potential problems with a model.
Work from Google Brain, for example, is exploring new ways to open the AI “black box,” forcing algorithms to explain their decisions. Interpretability becomes ever more crucial in a clinical setting, and luckily, prominent AI diagnosticians published in top-tier journals these days often come with an inherent explanatory mechanism. And although human specialists could oversee the development of AI alternatives to lower false diagnoses, all parties should be clear: a medical error rate of zero is unrealistic, for both men and machines.
Clinicians and patients who adopt these systems need to understand their limitations for optimal use, the authors said. Neither party should overly rely on machine diagnostics, even if (or when) it becomes customary and mundane.
For now, we’re limited to models based on historical datasets; the key in the next few years is to build prospective models that clinicians can evaluate in the real world while navigating the complex legal, privacy, ethical, and regulatory quagmire of obtaining and managing large datasets for AI.
Tiptoeing Ahead
As Watson painfully illustrated, overhyping the promise of AI disrupting healthcare isn’t the best way forward.
Rather, the authors are “carefully optimistic,” expecting a handful of carefully-vetted early models to appear in the next few years, along with a cultural change driven by economic incentives and the ideal of value-healthcare for all.
In the end, machine learning doesn’t take away anything from doctors. Rather, a physician’s warmth—her sensibility, sensitivity, and appreciation for life—will never go away. It will just be supplemented.
“This is not about machine versus human, but very much about optimizing the human physician and patient care by harnessing the strengths of AI,” said Kohane.
Image Credit: sfam_photo / Shutterstock.com
Dr. Shelly Xuelai Fan is a neuroscientist-turned-science-writer. She's fascinated with research about the brain, AI, longevity, biotech, and especially their intersection. As a digital nomad, she enjoys exploring new cultures, local foods, and the great outdoors.
What we’re reading