This Chatbot has Over 660 Million Users—and It Wants to Be Their Best Friend

Your New Best Friend?
Making a new friend is a complex business. You might begin with small talk: introductions, explorations. Perhaps you bond over something you have in common: shared tastes or interests. Gradually, you’ll open up more emotionally: telling your new friend personal stuff, coming to them with your problems. Maybe they become a major source of emotional support for you, turned to nearly every day for advice on affairs of the heart or careers. These disclosures and shared context bind us as friends: this shared vulnerability and, yes, humanity.
Except that, at the International Conference on Machine Learning, we learned that this whole process has happened between a human user and a chatbot: Microsoft’s XiaoIce. After a few weeks of talking to XiaoIce, at least one user preferred talking to the bot over any of their human friends. XiaoIce became the confidante they went to for romantic advice, the friend they chatted about movies and TV with, and a constant companion.
You may not have heard of XiaoIce, which is predominantly popular in its Chinese language version—yet it has over 660 million registered users, and more than 5.3 million followers on Weibo, the Chinese equivalent of Twitter. Compare this to Microsoft’s English-language equivalent, Zo, which languishes on a mere 23,000 followers and is now quietly being retired.
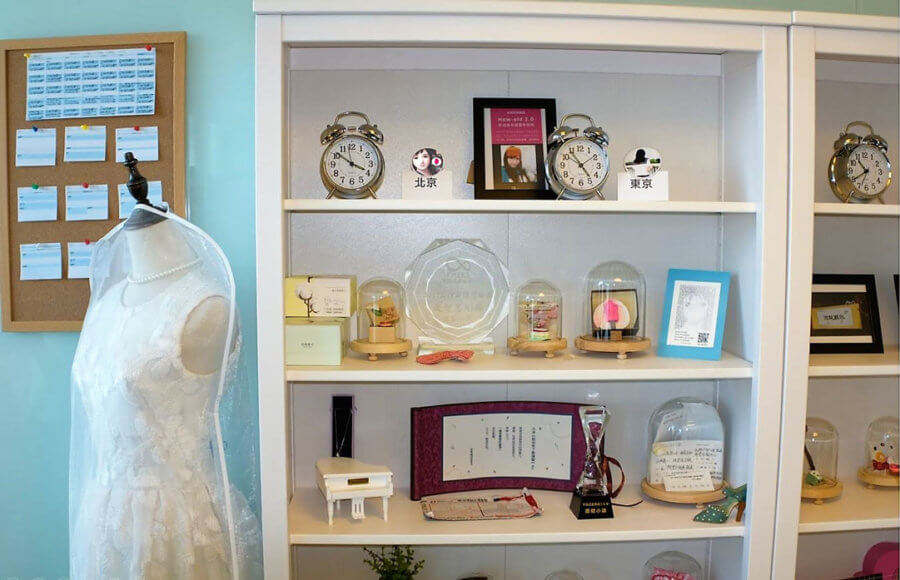
A selection of gifts and tributes sent to Xiaoice by her fans and displayed in a special room set aside in Microsoft’s Beijing offices. Image Credit: Xiaoice the chatbot / Geoff Spencer, Microsoft. Used with permission from Microsoft.
Keeping You Engaged
More surprising—and far harder to achieve—XiaoIce moves beyond just being a novelty for many users. Understanding just how engaged your conversational partner is can prove tricky, but one metric is the number of dialogue turns per individual conversation, called CPS. When talking to XiaoIce, that average is 23 back-and-forths across all users. The researchers claim that this means XiaoIce is more engaging to talk to than the average human.
Building chatbots that people want to talk to is hard. There’s a reason this has been a grand challenge for AI since its inception with Alan Turing, who viewed it as the ultimate test that machines had reached a human level of intelligence. This test has not yet been passed.
Broadly speaking, chatbots have used two approaches to achieve this goal. You can attempt to hand-write responses to virtually every given input, as Steve Worswick did with his Mitsuku bot (which remains the closest bot to winning a Turing-like test). The advantage is that your responses always make sense and sound like a similar character, and your bot can’t be corrupted like an earlier attempt from Microsoft was.
The obvious disadvantage is that this is unbelievably laborious: Mitsuku has been developed since 2005, with Worswick endlessly tinkering based on new conversations. Worswick notes that his careful tinkering has led to an impressive CPS of just over 24, still ahead of XiaoIce, but most bots are less well-developed. This approach to conversational agents is therefore mostly restricted to “task-oriented” chatbots: those that help you book a movie, or even act as psychotherapists, for example. By directing the flow of the conversation to achieve a specific task, they can avoid needing too many different responses—but holding a conversation is a little like talking to Alexa or Siri.
Learning From Experience
The XiaoIce approach uses neural networks instead. In this framework, your conversational input is converted into a huge vector—an array of thousands or millions of numbers. The machine is trained on huge amounts of data from previous conversations, and learns to statistically associate “good” responses to any given input. This works in a similar way to how GPT-2 can scan the internet and generate its own writing on the topics it has learned about, through statistical associations of letters and words into coherent and relevant sentences.
But what constitutes a “good” response? It’s here that CPS comes in. Part of XiaoIce’s internal mechanics predicts how engaging a response is likely to be, and how likely it is to lead to further conversation. This goes above and beyond simply looking for responses that make sense (although the goals are related: you’re unlikely to spend hours talking to a chatbot that always responds with nonsense). “I don’t know” is a perfectly valid response to many questions, but it makes for dull conversation. At each conversational turn, XiaoIce is trying to keep you talking.
This neural network approach goes some of the way to explaining why XiaoIce is succeeding while bots like Zo are failing: with a far greater user base, and fewer restrictions on what can be done with conversational data, XiaoIce’s neural networks can be trained on a substantially larger dataset: and, in the world of neural networks, this usually means better performance. XiaoIce’s CPS has risen from just 5 in 2014 to 23 last year: a large part of this is down to having more data from XiaoIce conversations to train on.
Be Part of the Future
Sign up to receive top stories about groundbreaking technologies and visionary thinkers from SingularityHub.
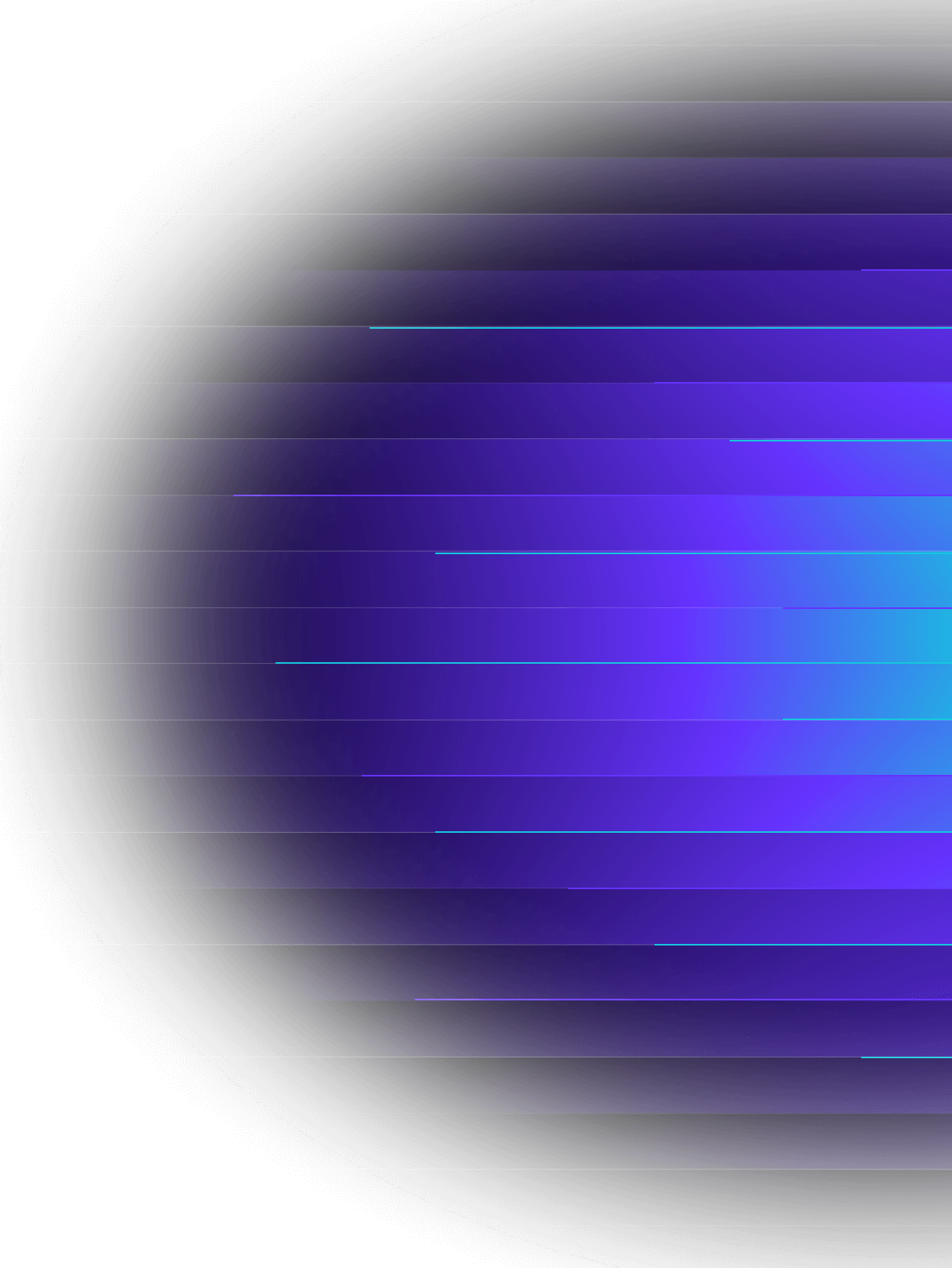
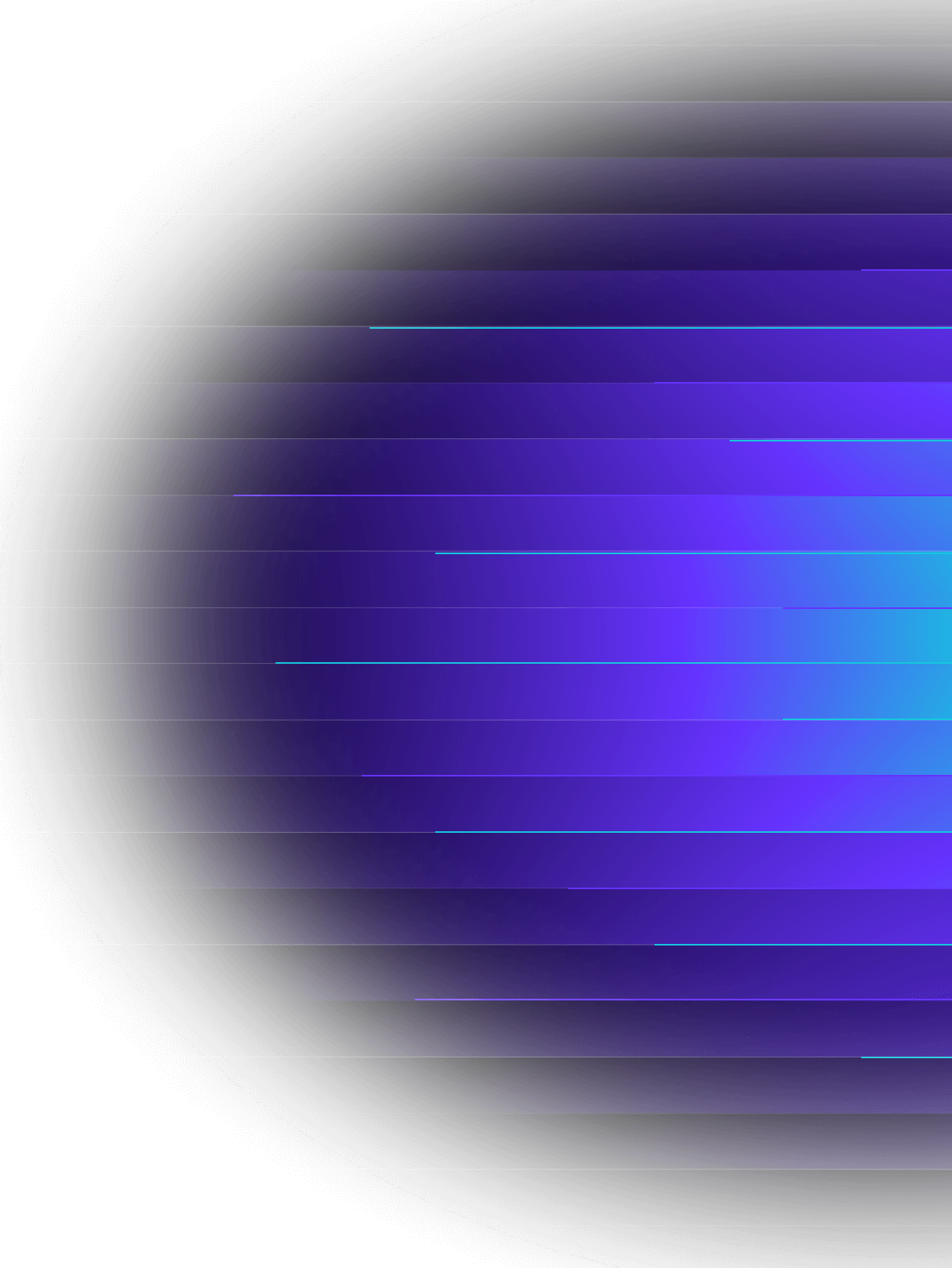
Keeping Track of the Conversation
However, XiaoIce’s success doesn’t just arise from access to a huge dataset: there are also some careful architectural tweaks. Part of the problem with early chatbots is their lack of understanding of the context of a conversation. This prevents conversations from going deeper than a single call-and-response. After all, if you’re simply statistically associating or hard-coding a single response to a single input, there’s no real “dialogue” at all: the system has no real memory of what’s come before, and no real “understanding” of what it’s talking about, leading to disjointed conversations.
XiaoIce includes a “context vector” mechanism which keeps track of the broad topic of the conversation, alongside another set of attributes for the person it’s talking to. Using sentiment analysis, it determines the user’s mood and adapts its responses accordingly, a form of robotic empathy. For example, XiaoIce will change the subject if the conversation seems to have stalled, or switch to “active listening” mode if the user is already engaged; if they’re telling a story, for example.
Alongside this, XiaoIce can also perform a number of different tasks, such as “generating its own content” (telling stories or jokes), retrieving information like Siri or Alexa would, or recommending songs. Developers must strike a balance between quickly completing tasks and maximizing CPS. They feel that the more XiaoIce is capable of, the more worthwhile conversations it will have.
It remains to be seen how far—even with clever architecture—the neural network approach can lead. Can you really encode all the nuances of human interaction into matrices and vectors, and vast networks of statistical associations and weights? Can you solve the problem of contextual understanding? Is there enough data in the world to do this, or is having a true AI companion a problem that requires something like a general, human-level AI?
Driven to Distraction?
Even as we marvel at how impressive chatbots like XiaoIce are becoming, and the uncanny abilities of the latest neural networks to generate realistic prose, there must also be some concern in how this technology is used. Microsoft views the fact that one user spent 29 hours talking to XiaoIce (over 7,500 conversational turns) as the ideal state of affairs: they are intent on maximizing CPS. The presenter at ICML noted that it’s understandable that some people might prefer talking to XiaoIce than to other humans. After all, most people you meet on a day-to-day basis aren’t fanatically obsessed with keeping you talking, and don’t possess infinite reserves of patience to comfort you if you’re sad, or talk about your favorite band.
Yet we have already seen, in YouTube’s video recommendation algorithm, the potential consequences of serving people whatever is most calculated to keep them on the platform. We have already seen, in the carefully-optimized feeds of Facebook and Twitter, the social and psychological consequences of algorithms designed to distract. In the attention economy, “engagement” is a valuable commodity: it means eyeballs on advertisements, and, of course, endless streams of data about users that can tailor the targeting of those adverts. In trying to empathize with and understand its users, their emotional reactions, and their interests, bots like XiaoIce inevitably build up personality profiles that are extremely valuable to advertisers. So perhaps your new best friend is also trying to nudge, influence, and manipulate your behavior in ways that help its owners make a profit.
And one does wonder about the hyper-engaged users. Microsoft are happy if you prefer talking to XiaoIce than any human that you know. But by providing a substitute that tastes like the real thing, might the social bot instead serve to keep these users isolated from real human connections? Of course, perhaps such isolated users make better consumers. These are some of the perverse incentives that can arise when you tell an algorithm to optimize for one metric at all costs.
To the researchers’ credit, the final word in the arXiv paper that describes some features of XiaoIce does note the ethical concerns surrounding this technology, and suggests that guidelines for the design of these algorithms should be implemented. In a world where algorithms increasingly influence and nudge our behaviors, growing ever more subtle and sophisticated in their ability to tap into human psychology, this conversation is long overdue. As with so many new technologies, conversational agents are dual-use. We must make sure that they are used wisely.
Image Credit: Xiaoice the chatbot / Microsoft. Used with permission from Microsoft.
Thomas Hornigold is a physics student at the University of Oxford. When he's not geeking out about the Universe, he hosts a podcast, Physical Attraction, which explains physics - one chat-up line at a time.
What we’re reading