How Old Is Your Brain? This AI Can Tell You

Delaying “brain age” may sound like the latest quick-fix gimmick on a late-night infomercial, but the science underlying the concept is very real.
Rather than reflecting the average functional state of your chronological age, brain age looks at how well your brain is aging relative to how many birthdays you’ve celebrated. We all know people that seem sharper and act much younger than their age—that incredulous moment when you realize the 40-year-old you’ve been chatting with on the plane is actually a grandma in her 70s. Brain age, as a concept, hopes to capture the biological intricacies behind that cognitive dissociation.
It’s not just pure academic fun, either. Longevity researchers have increasingly realized that how long you’ve lived isn’t the best predictor of overall health. A precise and easy way to gauge a person’s true biological brain age could be an invaluable canary in the coal mine—if you know your brain is aging faster than it should, you’re empowered by that knowledge to intervene early.
This week, an ambitious study in Nature Neuroscience married three disparate fields—neuroscience, longevity, and machine learning—into a single algorithm that can predict a person’s brain age based purely on MRI scans.
Using data from nearly 50,000 people with ages spanning 8 decades, the study is a first to tease out how common brain disorders, such as depression and autism, affect brain aging. What’s more, the team dug deep down into human genomic data from UK Biobank, pinpointing sets of genes related to neurological disorders that especially accelerate brain aging.
“We reveal that genes involved in apparent brain aging in healthy individuals overlap with genes that we know to be associated with common brain disorders,” said study author Tobias Kaufmann at the University of Oslo in Norway.
The immediate use of such a “brain age gap” is as a biomarker for brain aging, which can help doctors make informed decisions about their aging patients.
But aside from the study’s findings, perhaps its most significant contribution is its validation of an interdisciplinary approach “only made possible by the ability to study brain scans from a very large number of people” across scanners, sites, and settings, said Dr. Janine Bijsterbosch at Washington University Medicine School in Saint Louis, Missouri. Bijsterbosch was not involved in the study, but wrote an accompanying perspective piece.
Translation? Data from individual labs are no longer adequate to hunt down tiny, intricate, but powerful markers of brain aging—or other neurological measures and health insights. To better unveil the mysteries of our brains at a population level, across racial and socioeconomic divides, it pays to recognize—and utilize—this “power in numbers” across research institutions.
Brain Age and Healthspan
In late 2015, a series of expert commentaries in Nature Medicine consolidated an emerging trend in longevity research. Rather than trying to lengthen lifespan, the current focus should be more on extending healthspan—how long you can live without disease, or how long you can delay the onset of common age-related illnesses.
This immediately poses a problem: how do you measure a person’s “true” biological age? It’s a yet unsolved conundrum.
But for the brain, one marker is leading the flock: the brain age gap, or the difference between a person’s chronological age and brain age. A large gap in either direction suggests that a person’s brain is aging faster or slower than the norm.
The molecular orchestra that controls how fast a brain matures and changes throughout lifespan plays a significant role in brain structure, which can be measured using MRI. Similarly, the biological dance that determines the physical connections of neural circuits also underlies brain disorders like autism, schizophrenia, bipolar, or depression.
This led the team to ask: is there a way to use MRI scans to gauge a person’s brain age gap? How would it change with different mental disorders? And can we link brain age to specific genes, uncovering those that either accelerate and delay brain aging?
A Giant Study
Kaufmann and his colleagues aren’t the first to tackle the problem, but they’re certainly the most ambitious. Previous studies have been “small-scale,” they explained, in that they only looked at a limited age range and usually focused on a single mental disorder, with a maximum of a few hundred people. The results can’t provide a whole, dynamic picture of structural brain changes over an entire lifespan.
Because no single lab can provide the data they need, the team decided to aggregate MRI scan data from several sites, obtained by different MRI scanners under different settings. In the past, this was nothing short of crazy, because these variations make apple-to-apple comparisons between images extremely difficult. Using a cooking analogy, it’s like trying to consolidate hundreds of thousands of hand-scribbled recipes of the same dish, each written with a personal format using a range of units and abbreviations, and trying to decipher an average “baseline” recipe to adequately judge all others.
The team relied on a series of advanced data methods to wrangle data from 45,615 people into standardized sets—a task that took considerable effort, time, and trial and error. As a sanity check, they then included this information in their machine learning algorithm to double check for potential normalization errors. Next, using data from over 35,00 healthy individuals 3 to 89 years old, they trained the AI to predict a normal brain aging trajectory.
Be Part of the Future
Sign up to receive top stories about groundbreaking technologies and visionary thinkers from SingularityHub.
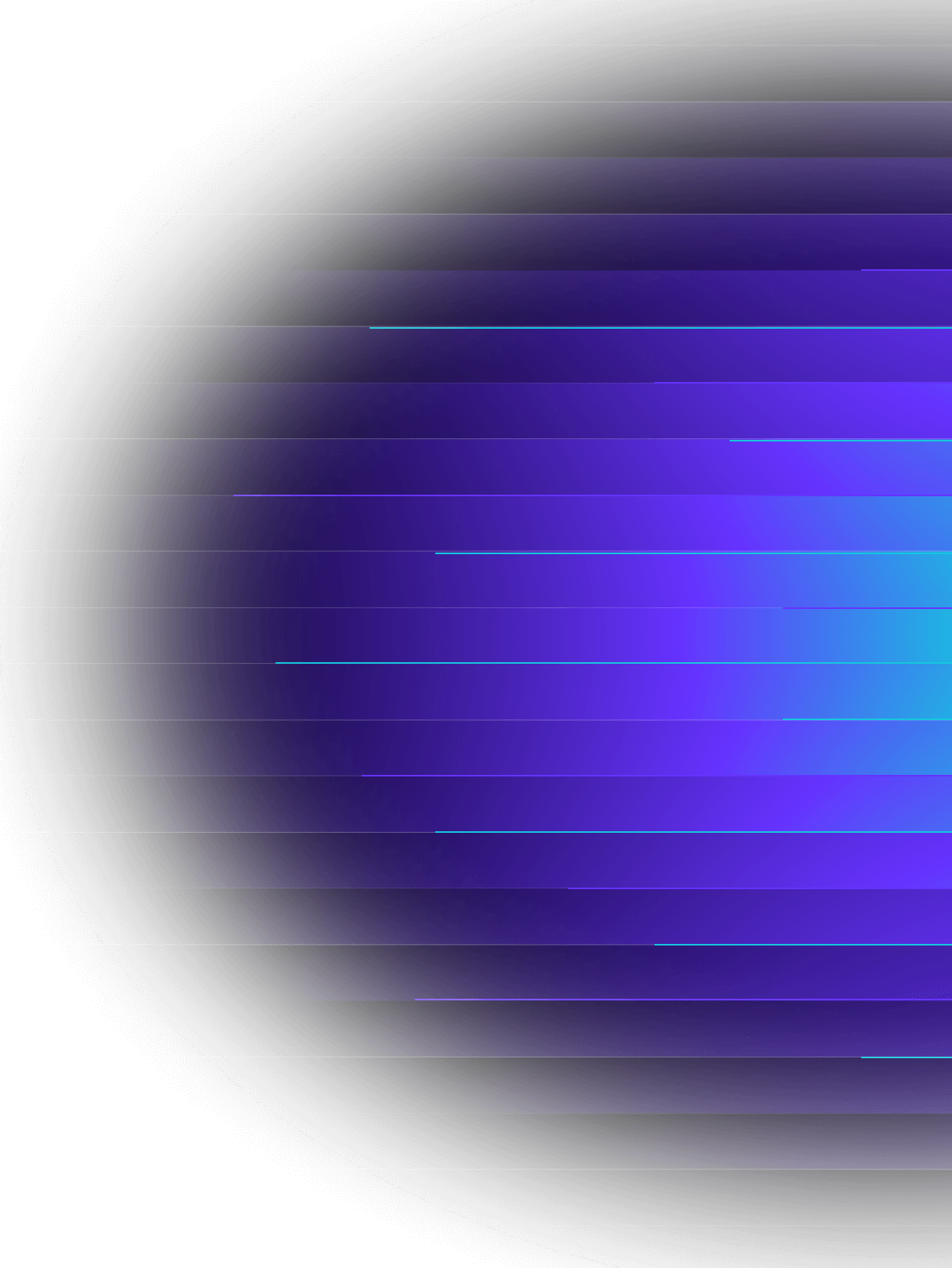
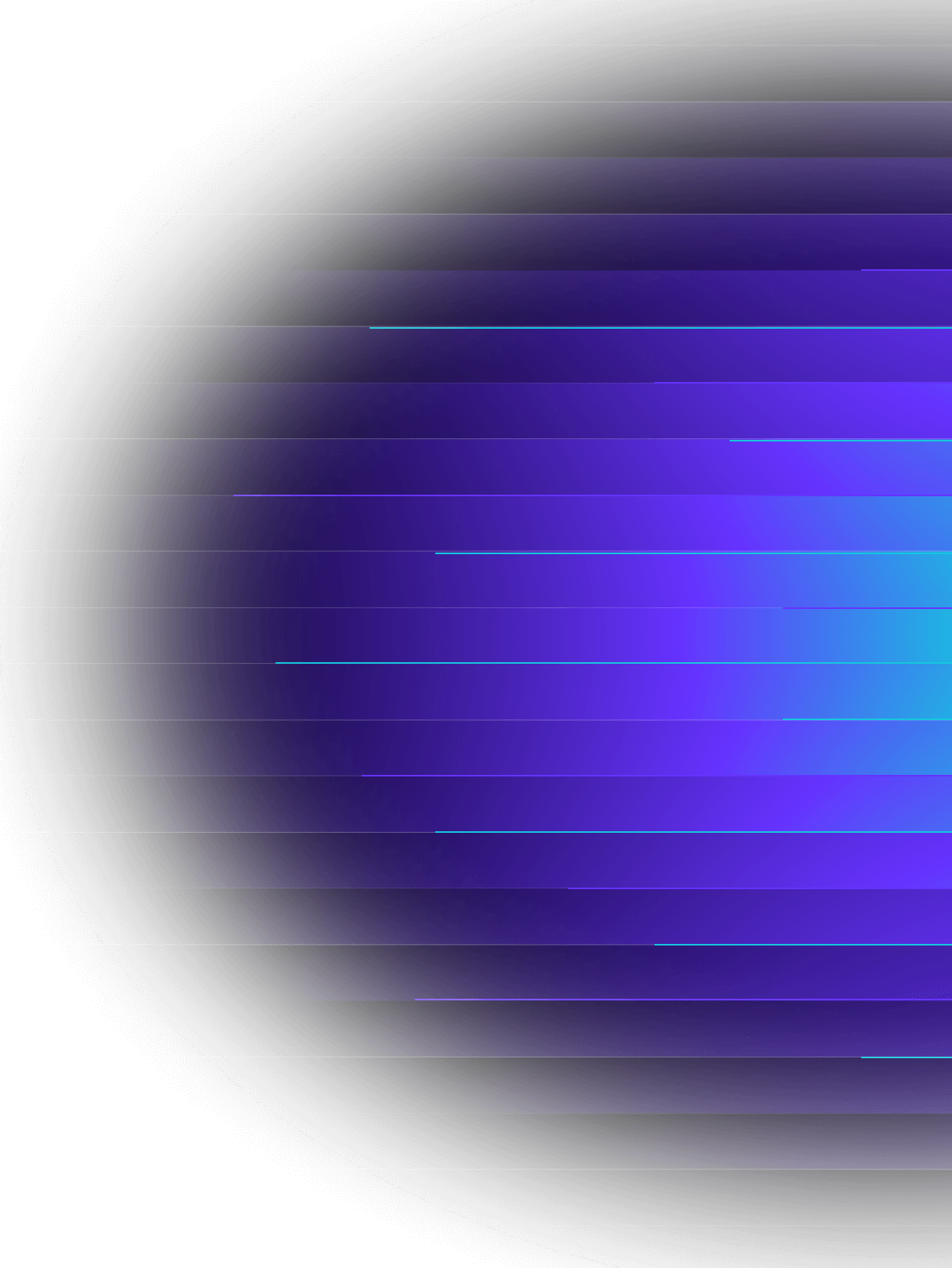
The algorithm was then validated with data from an additional 4,353 healthy people. Finally, the team compared brain scans from nearly 5,800 people with various brain disorders, matching each person’s brain age to the general trajectory.
Several insights emerged. The biggest brain age gaps were found in severe mental disorders, including schizophrenia, multiple sclerosis, and dementia. In contrast, developmental brain disorders such as autism and ADHD didn’t seem to particularly affect brain age. Full brain changes aside, the team also found that brain regions that significantly contributed to the brain age gap were the ones already implicated in that particular mental disorder. For example, in Alzheimer’s disease the structure of regions under the cortex slowly withers—they’re also the ones triggering the brain age gap as measured by the algorithm.
That’s an important validation, the team said. It shows that the AI can condense information from a large number of brain images into an interpretable score, without completely losing information about individual brain regions. In other words, some disorders may cause one brain region to age faster than others. The AI can decipher these differences and guide potential treatments.
A Genetic Link
An additional bonus of the aggregated dataset is that it included genetic information linked to the brain scans. Accelerated brain aging could be the consequence of being dealt a bad genetic hand, aggravated by detrimental environments or lifestyle choices. Analyzing genes is one way to begin exploring factors that influence the variations in brain aging trajectories, the authors said.
Perhaps unsurprisingly, an analysis showed that the brain age gap is at least partially heritable. The team also identified a handful of genes that seem to contribute to both brain age gap and brain disorder—that is, they each packed a one-two punch depending on the individual.
“Genetic variants associated with brain age gaps in healthy individuals partly overlap with those observed in autism, ADHD…[and others],” said the team. “These results suggest shared molecular genetic mechanisms between brain age gaps and brain disorders.”
Individual Brain Age
The AI is a first step in helping determine brain age in an individual. Going from averaged results to individual scans is difficult, said Bijsterbosch, because MRI scans are relatively noisy with large variability between people. More research is needed, but the study, given its large size, is a solid foundation.
Eventually, the hope is to predict a person’s brain age gap, based on their genetics, before the onset of high-risk brain disorders, and track disease progression over time to help adjust their treatments.
“We are still a very long way off from using the brain age gap in this way,” said Bijsterbosch. But with multiple large-scale biomarker studies already underway, this sterling example of a neuroscience-AI crossover is just the beginning.
Image Credit: christitzeimaging.com / Shutterstock.com
Dr. Shelly Xuelai Fan is a neuroscientist-turned-science-writer. She's fascinated with research about the brain, AI, longevity, biotech, and especially their intersection. As a digital nomad, she enjoys exploring new cultures, local foods, and the great outdoors.
What we’re reading