People Trust Deepfake Faces Generated by AI More Than Real Ones, Study Finds

Share
The proliferation of deepfake technology is raising concerns that AI could start to warp our sense of shared reality. New research suggests AI-synthesized faces don't simply dupe us into thinking they're real people, we actually trust them more than our fellow humans.
In 2018, Nvidia wowed the world with an AI that could churn out ultra-realistic photos of people that don’t exist. Its researchers relied on a type of algorithm known as a generative adversarial network (GAN), which pits two neural networks against each other, one trying to spot fakes and the other trying to generate more convincing ones. Given enough time, GANS can generate remarkably good counterfeits.
Since then, capabilities have improved considerably, with some worrying implications: enabling scammers to trick people, making it possible to splice people into porn movies without their consent, and undermining trust in online media. While it’s possible to use AI itself to spot deepfakes, tech companies’ failures to effectively moderate much less complicated material suggests this won’t be a silver bullet.
That means the more pertinent question is whether humans can spot the difference, and more importantly how they relate to deepfakes. The results from a new study in PNAS are not promising—researchers found that peoples’ ability to detect fakes was no better than a random guess, and they actually rated the made-up faces as more trustworthy than the real ones.
“Our evaluation of the photorealism of AI-synthesized faces indicates that synthesis engines have passed through the uncanny valley and are capable of creating faces that are indistinguishable—and more trustworthy—than real faces,” the authors wrote.
To test reactions to fake faces, the researchers used an updated version of Nvidia’s GAN to generate 400 of them, with an equal gender split and 100 faces each from four ethnic groups: Black, Caucasian, East Asian, and South Asian. They matched each of these with real faces pulled from the database that was originally used to train the GAN, which had been judged to be similar by a different neural network.
They then recruited 315 participants from the Amazon Mechanical Turk crowdsourcing platform. Each person was asked to judge 128 faces from the combined dataset and decide if they were fake or not. They achieved an accuracy rate of just 48 percent, actually worse than the 50 percent you should get from a random guess.
Deepfakes often have characteristic defects and glitches that can help people single them out. So the researchers carried out a second experiment with another 219 participants where they gave them some basic training in what to look out for before getting them to judge the same number of faces. Their performance improved only slightly, to 59 percent.
In a final experiment, the team decided to see if more immediate gut reactions to faces might give people better clues. They decided to see whether trustworthiness—something we typically decide in a split second based on hard-to-pin-down features—might help people make better calls. But when they got another 223 participants to rate the trustworthiness of 128 faces, they found people actually rated the fake ones 8 percent more trustworthy, a small but statistically significant difference.
Given the nefarious uses deepfakes can be put to, that is a worrying finding. The researchers suggest that part of the reason why the fake faces are rated more highly is because they tend to look more like average faces, which previous research has found people tend to trust more. This was born out by looking at the four most untrustworthy faces, which were all real, and the three most trustworthy, which were all fake.
Be Part of the Future
Sign up to receive top stories about groundbreaking technologies and visionary thinkers from SingularityHub.
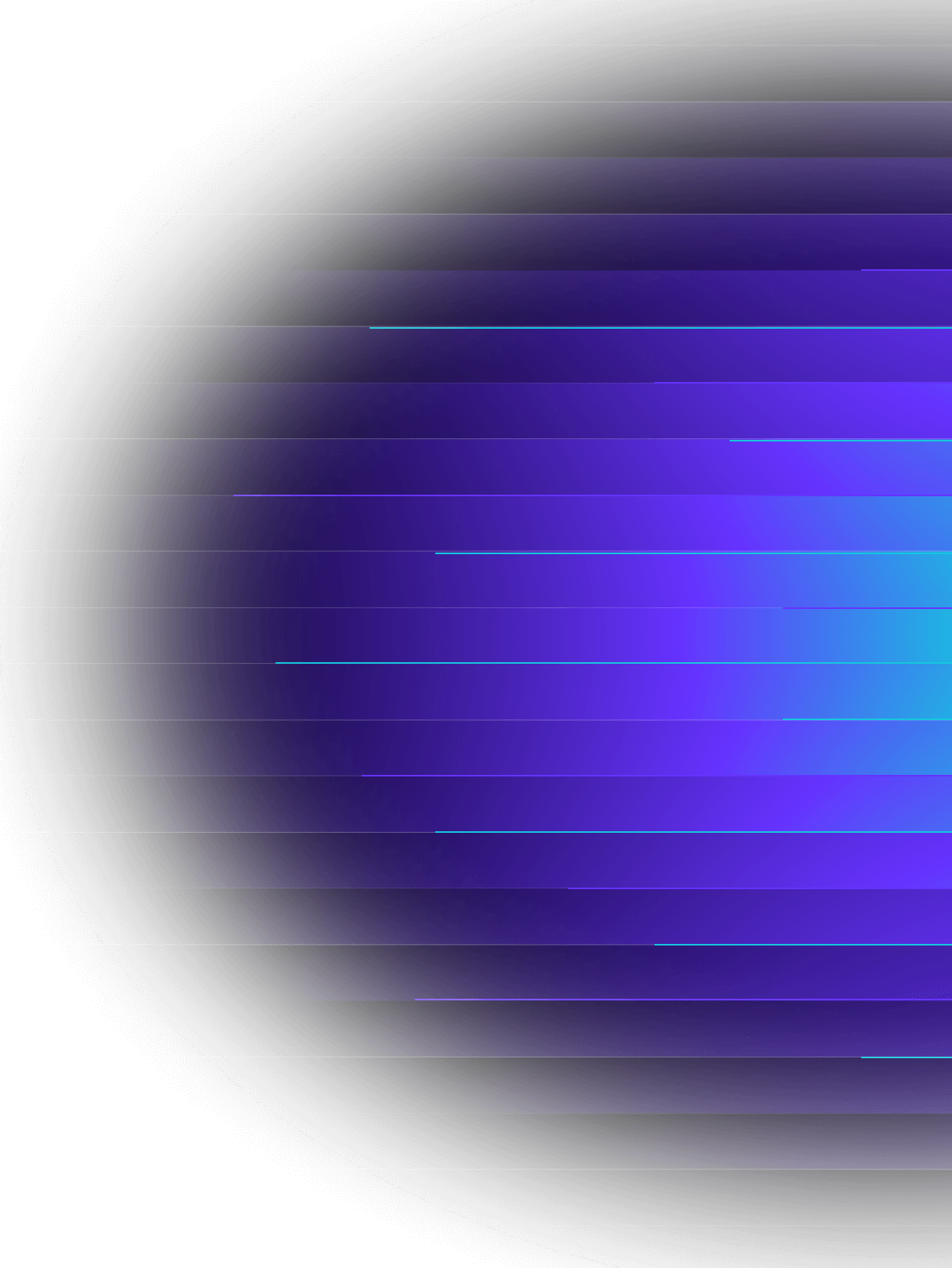
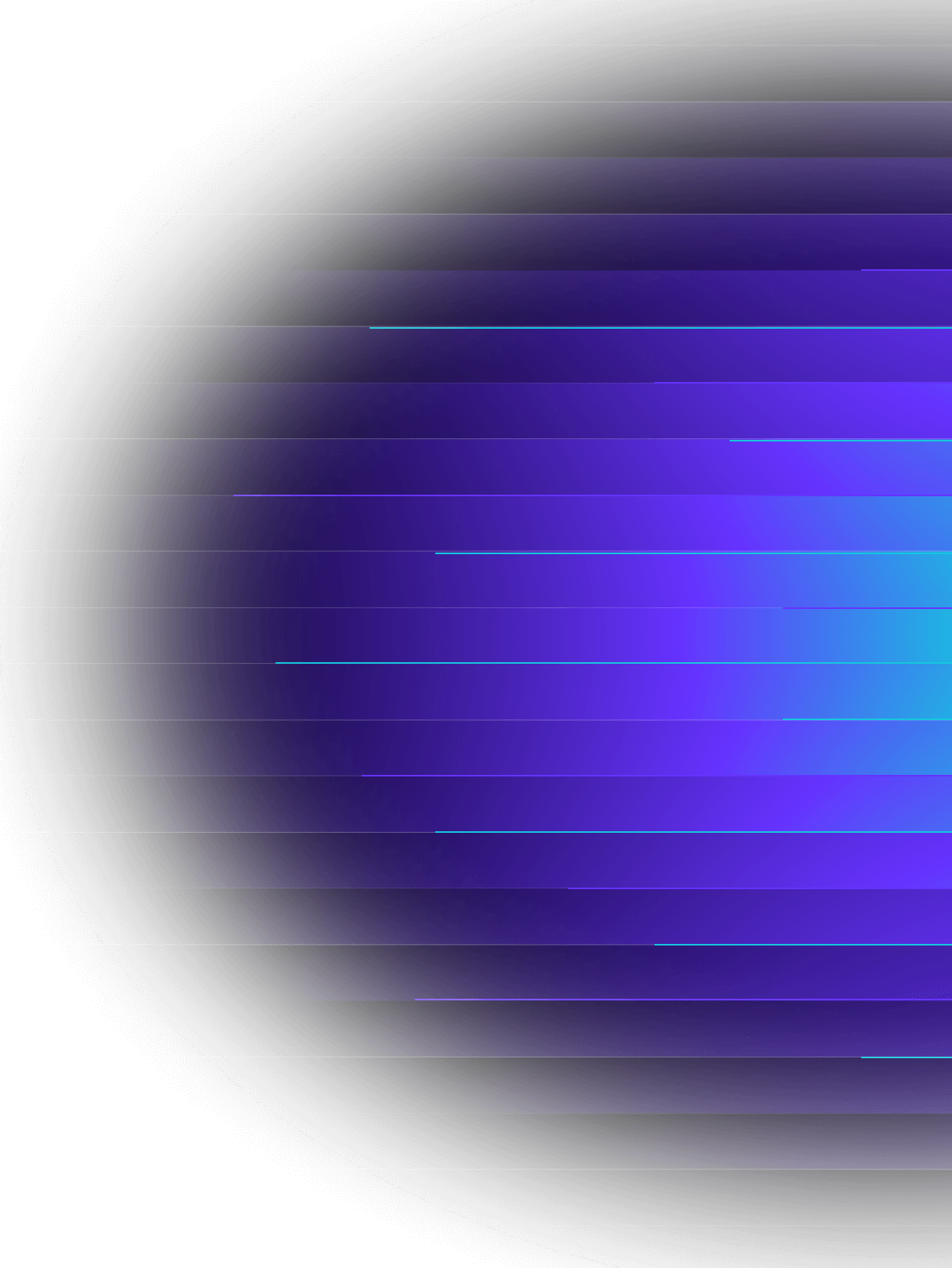
The researchers say their findings suggest that those developing the underlying technology behind deepfakes need to think hard about what they’re doing. An important first step is to ask themselves whether the benefits of the technology outweigh its risks. The industry should also consider building in safeguards, which could include things like getting deepfake generators to add watermarks to their output.
“Because it is the democratization of access to this powerful technology that poses the most significant threat, we also encourage reconsideration of the often laissez-faire approach to the public and unrestricted releasing of code for anyone to incorporate into any application,” the authors wrote.
Unfortunately though, it might be too late for that. Publicly-available models are already capable of producing highly convincing deepfakes, and it seems unlikely that we’ll be able to put the genie back in the bottle.
Image Credit: geralt / 23929 images
Looking for ways to stay ahead of the pace of change? Rethink what’s possible. Join a highly curated, exclusive cohort of 80 executives for Singularity’s flagship Executive Program (EP), a five-day, fully immersive leadership transformation program that disrupts existing ways of thinking. Discover a new mindset, toolset and network of fellow futurists committed to finding solutions to the fast pace of change in the world. Click here to learn more and apply today!
Related Articles

Meta’s New AI Translates Speech in Real Time Across More Than 100 Languages

What Is an AI Agent? A Computer Scientist Explains the Next Wave of AI Tools
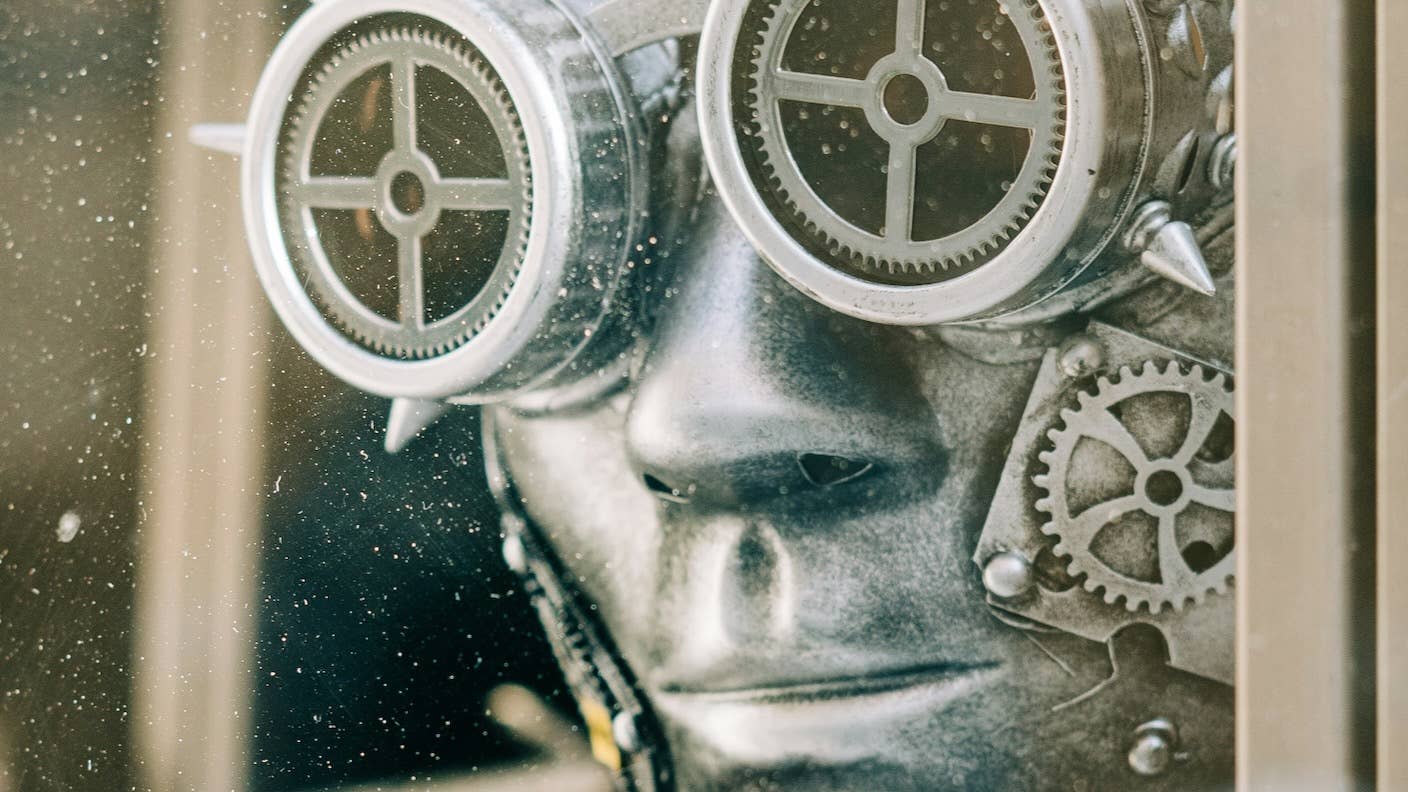
Is 2025 the Year AI Agents Take Over? Industry Bets Billions on AI’s Killer App
What we’re reading