This AI Can Design Complex Proteins Perfectly Tailored to Our Needs

Building proteins with AI is like furnishing a house.
There are two main strategies. One is the IKEA approach: you buy pre-made pieces that easily snap together, but can only hope the furniture somewhat fits your space. While relatively simple, you have no control over the dimensions or functions of the final product.
The other way starts with a vision and design perfectly tailored to your needs. But the hard part is finding—or building—individual pieces for the custom design.
The same two methods apply to engineering protein complexes using AI. Similar to a cabinet, protein complexes are made of multiple sub-units that intricately bind together. These mega structures—with shapes ranging from a twenty-sided die to tunnels that open and close—form the foundation of our metabolism, immune defenses, and brain functions.
Previous attempts at shaping protein architectures mostly used the IKEA approach. It’s revolutionary: AI-based designs have already generated COVID vaccines at lightning speed. While powerful, the approach is limited by available protein “building blocks.”
This month, a team led by Dr. David Baker from the University of Washington took protein design to a new custom level. Starting with specific dimensions, shapes, and other properties, the team tapped into a machine learning algorithm to build protein complexes tailored to specific biological responses.
In other words, rather than the usual bottom-up method, they went top-down.
One design, for example, is a 20-sided shell that mimics the outer protective layer of viruses. When dotted with immune-stimulating proteins from the flu virus, the AI-designed protein shell sparked an immune response in mice that outperformed the latest vaccine candidates in clinical trials.
The AI isn’t just for vaccines. The same strategy could build more compact and efficient carriers for gene therapies or carry antibodies and other drugs that need extra protection from being immediately broken down in the body.
But more broadly, the study shows that it’s possible to design massively complex protein architectures starting from an overall vision, rather than working with the biological equivalent of two-by-four boards.
“It’s astounding that the team could do this,” said Dr. Martin Noble at Newcastle University, who was not involved in the work. “It takes evolution billions of years to design single proteins that fold just right, but this is another level of complexity, to fold proteins to fit so well together and make closed structures.”
Evolution at Warp Speed
At the heart of the new work is reinforcement learning. You’ve probably heard of it. Loosely based on how the brain learns through trial and error, reinforcement learning powers multiple AI agents that have taken the world by storm. Perhaps the best known is AlphaGo, the DeepMind brainchild that triumphed over the human world champion in the board game Go. More recently, reinforcement learning has been speeding progress in self-driving cars and even developing better algorithms by streamlining fundamental computations.
In the new study, the team tapped into a type of reinforcement learning algorithm called the Monte Carlo tree search (MCTS). While sounding like a casino move, it’s a popular reinforcement learning strategy that searches for optimized decisions.
Picture the algorithm as a tree of your life decisions. We’ve likely all wondered how our lives would be if we made a different choice at some point. If you draw out those alternative decisions as a timeline—voilà, you have a decision tree, with each combination of branches leading to a different outcome.
MCTS, then, is a bit like the game of life. Choices are selected at each branch randomly and followed down that path of the tree. Once it reaches the final outcome, it feeds back up the tree to increase the probability of your desired solution. It’s like exploring the multiverse in Everything, Everywhere, All At Once—but instead of life choices, here it’s for designing proteins.
To start, the team fed the MCTS algorithm millions of protein fragments with specific building goals. The fragment amounts were carefully weighed: a smaller number at each calculation step speeds up the AI’s learning process and increases the diversity of the final protein. But more pieces also cause computation time and energy use to skyrocket. Balancing the dilemma, the team built several protein structural elements as a starting point to begin the protein design search.
Like fumbling with digital Play-Doh, the algorithm then twisted or bent protein fragments to see if they passed the overall geometric constraints of the final protein—including its backbone and its “attachment points” to help the fragments self-assemble. If the simulations got the thumbs up, their computational pathways were “boosted” in the algorithm. Rinse and repeat tens of thousands of times, and the program can hone in on optimal individual parts for a certain design.
While it sounds like a massive undertaking, the algorithm was highly efficient. Each iteration on average took only tens of milliseconds, the team explained.
Be Part of the Future
Sign up to receive top stories about groundbreaking technologies and visionary thinkers from SingularityHub.
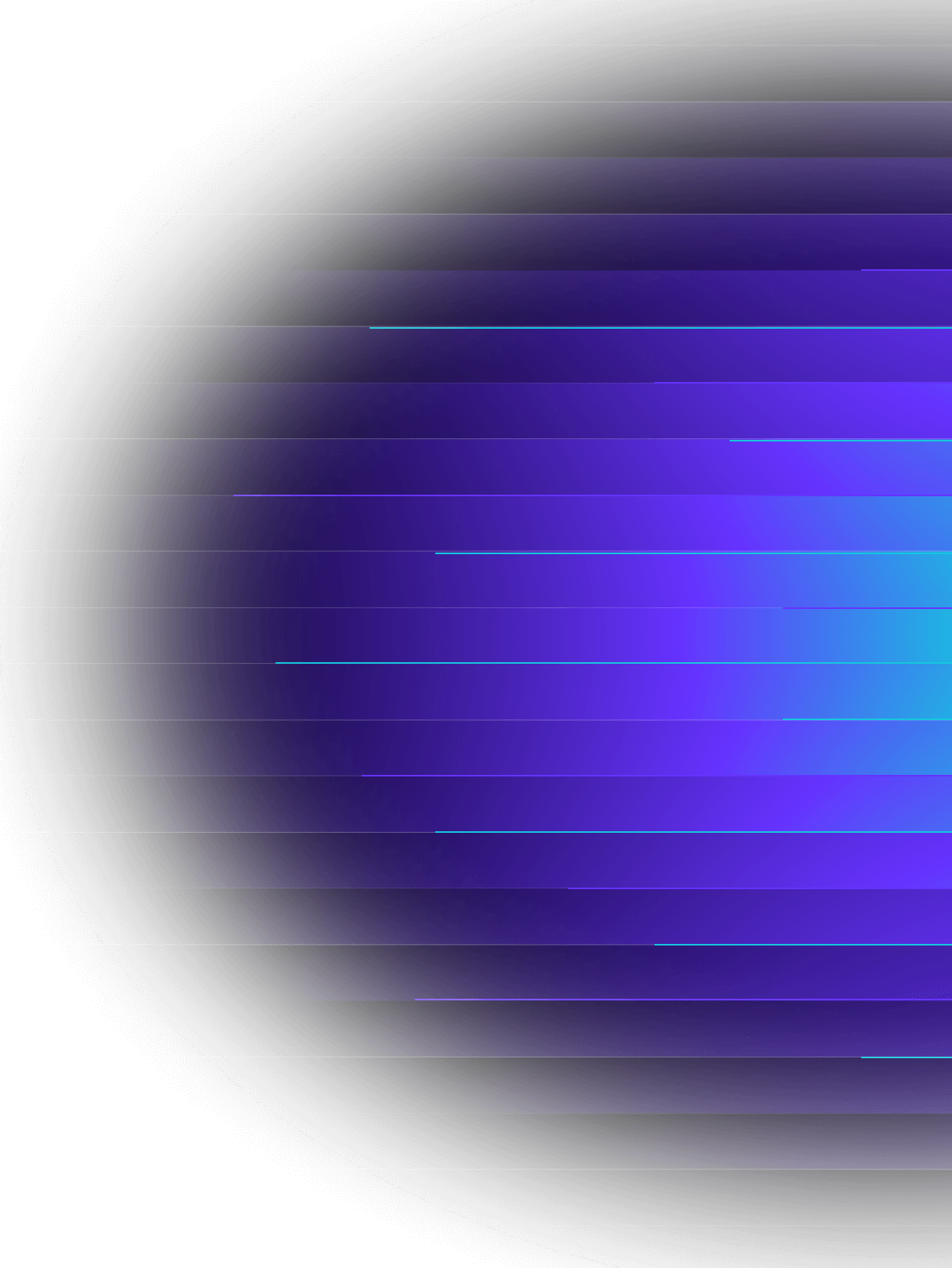
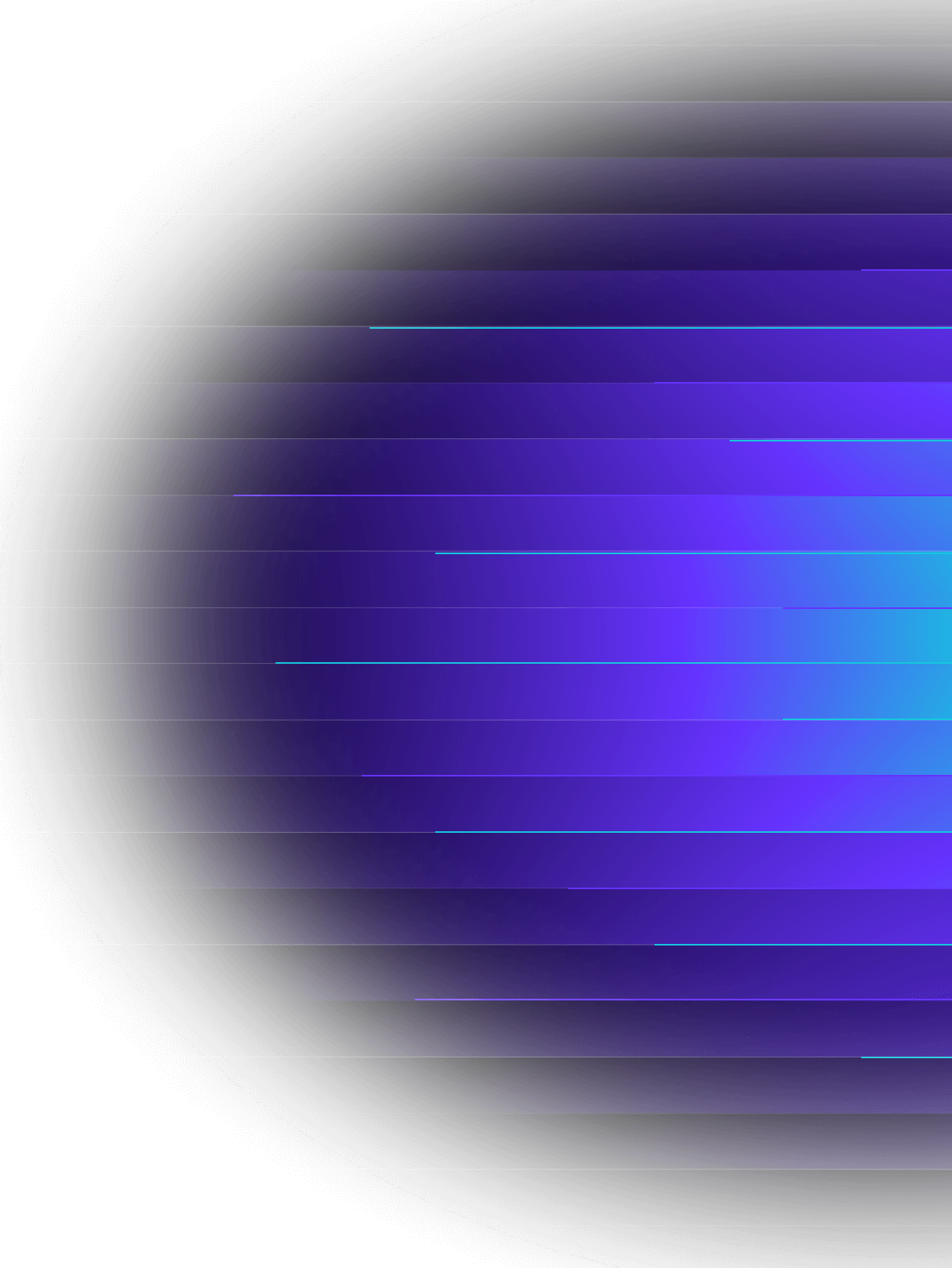
Proteins on Demand
In the end, the team had a powerful algorithm that—like an architect—designed proteins based on custom needs. In one test, the AI made a range of protein structures from prisms to pyramids and letters of the alphabet, with each filling a specific space as required.
“Our approach is unique because we use reinforcement learning to solve the problem of creating protein shapes that fit together like pieces of a puzzle. This simply was not possible using prior approaches and has the potential to transform the types of molecules we can build,” said study author Isaac Lutz.
But how do the AI designs translate to real life?
As a proof of concept, the team made hundreds of proteins in the lab to test for fidelity. Using an electron microscope, the AI-designed proteins were almost identical to the predicted blueprints at the atomic scale.
One design standout was a hollow shell made with dozens of protein pieces. Called a capsid, the structure resembles the protective protein layer for viruses—one often used as a guide to generate vaccines. Unlike previous iterations, the AI-generated shells were densely packed with multiple attachment points. Like wall anchors, these can help the structures dock onto cells or better package material—drugs, gene therapies, or other biological materials—inside the scaffold.
At roughly 10 nanometers, these nano-capsids are “considerably smaller than most viral” ones, the team explained.
The petite sizing came with a big medicinal punch. In one test, the team dotted the capsids with 60 copies of a protein that helps stimulate blood vessel growth in human cells from the umbilical veins. The AI-made protein bubble outperformed a previous nanoparticle more than 10-fold. This “opens up potential applications…for diabetes, brain injuries, strokes, and other cases where blood vessels are at risk,” said study author Dr. Hannele Ruohola-Baker.
Another experiment took full advantage of the dense attachment points on the 20-sided shell, transforming the capsid into an efficient vaccine. Here, the team fused a flu protein HA (influenza hemagglutinin) to the nano-capsid and injected it into mice. Compared to a similar but much larger vaccine design already in clinical trials, the AI-designed solution sparked a heftier immune response.
For now, the AI is still in its early stages. But as the past two years have shown, it’ll rapidly evolve. The 20-sided shell and other structures “are distinct from any previously designed or naturally-occurring structures,” said the team. Thanks to their small size but large carrying capacity, they can potentially tunnel inside the cell nucleus—which houses DNA—and efficiently shuttle gene editing components.
“Its potential to make all kinds of architectures has yet to be fully explored,” said study author Dr. Shunzhi Wang.
Image Credit: Ian Haydon/ UW Medicine Institute for Protein Design
Dr. Shelly Xuelai Fan is a neuroscientist-turned-science-writer. She's fascinated with research about the brain, AI, longevity, biotech, and especially their intersection. As a digital nomad, she enjoys exploring new cultures, local foods, and the great outdoors.
What we’re reading