This Graphene-Based Brain Implant Can Peer Deep Into the Brain From Its Surface

Finding ways to reduce the invasiveness of brain implants could greatly expand their potential applications. A new device tested in mice that sits on the brain’s surface—but can still read activity deep within—could lead to safer and more effective ways to read neural activity.
There are already a variety of technologies that allow us to peer into the inner workings of the brain, but they all come with limitations. Minimally invasive approaches include functional MRI, where an MRI scanner is used to image changes of blood flow in the brain, and EEG, where electrodes placed on the scalp are used to pick up the brain’s electrical signals.
The former requires the patient to sit in an MRI machine though, and the latter is too imprecise for most applications. The gold standard approach involves inserting electrodes deep into brain tissue to obtain the highest quality readouts. But this requires a risky surgical procedure, and scarring and the inevitable shifting of the electrodes can lead to the signal degrading over time.
Another approach involves laying electrodes on the surface of the brain, which is less risky than deep brain implants but provides greater accuracy than non-invasive approaches. But typically, these devices can only read activity from neurons in the outer layers of the brain.
Now, researchers have developed a thin, transparent surface implant with electrodes made from graphene that can read neural activity deep in the brain. The approach relies on machine learning to uncover relationships between signals in outer layers and those far below the surface.
“We are expanding the spatial reach of neural recordings with this technology,” Duygu Kuzum, a professor at UC San Diego who led the research, said in a press release. “Even though our implant resides on the brain’s surface, its design goes beyond the limits of physical sensing in that it can infer neural activity from deeper layers.”
The device itself is made from a thin polymer strip embedded with a dense array of tiny graphene electrodes just 20 micrometers across and connected by ultra-thin graphene wires to a circuit board. Shrinking graphene electrodes to this size is a considerable challenge, say the authors, as it raises their impedance and makes them less sensitive. They got around this by using a bespoke fabrication technique to deposit platinum particles onto the electrodes to boost electron flow.
Crucially, both the electrodes and the polymer strip are transparent. When the team implanted the device in mice, the researchers were able to shine laser light through the implant to image cells deeper in the animals’ brains. This made it possible to simultaneously record electrically from the surface and optically from deeper brain regions.
In these recordings, the team discovered a correlation between the activity in the outer layers and inner ones. So, they decided to see if they could use machine learning to predict one from the other. They trained an artificial neural network on the two data streams and discovered it could predict the activity of calcium ions—an indicator of neural activity—in populations of neurons and single cells in deeper regions of the brain.
Be Part of the Future
Sign up to receive top stories about groundbreaking technologies and visionary thinkers from SingularityHub.
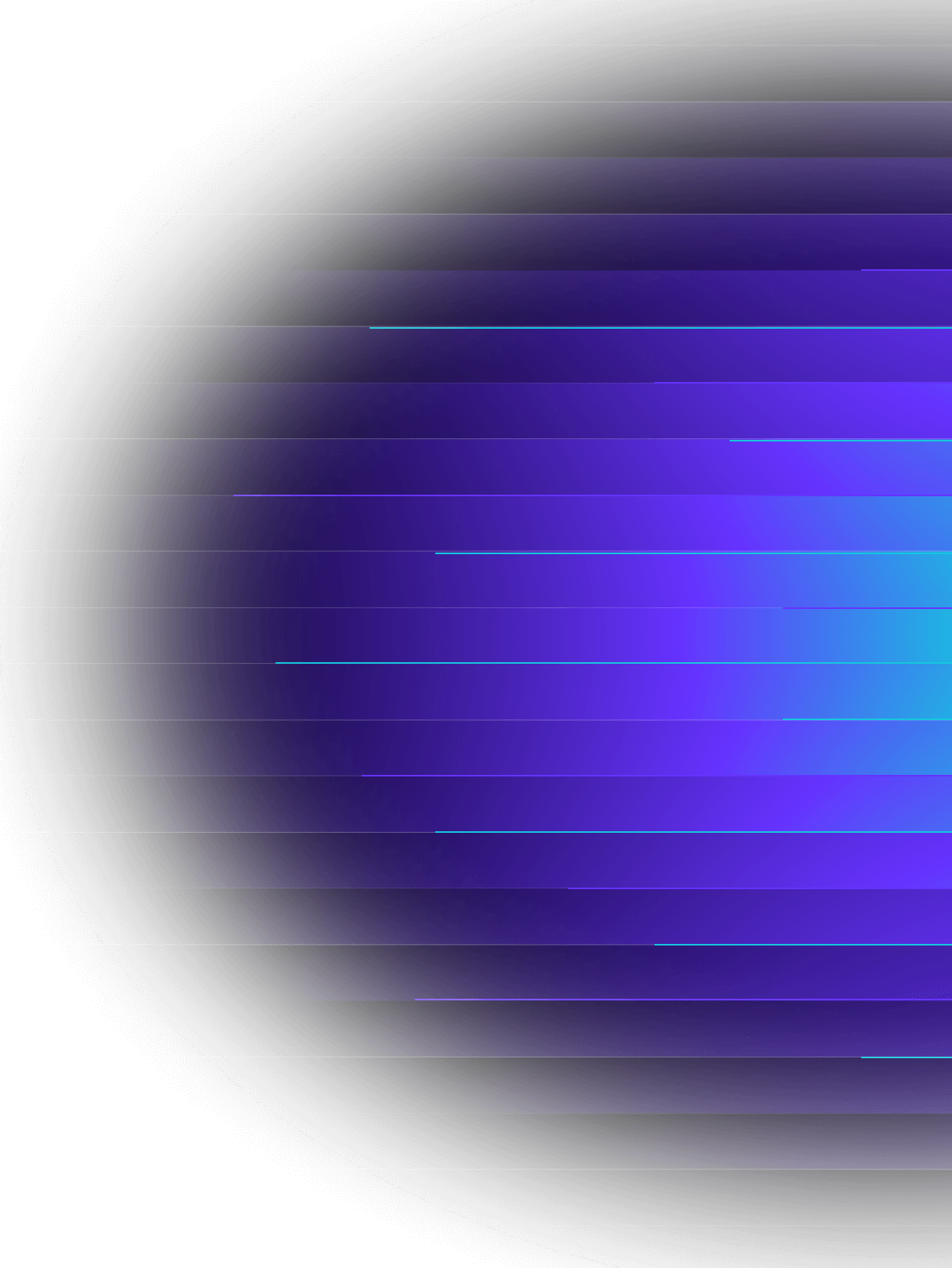
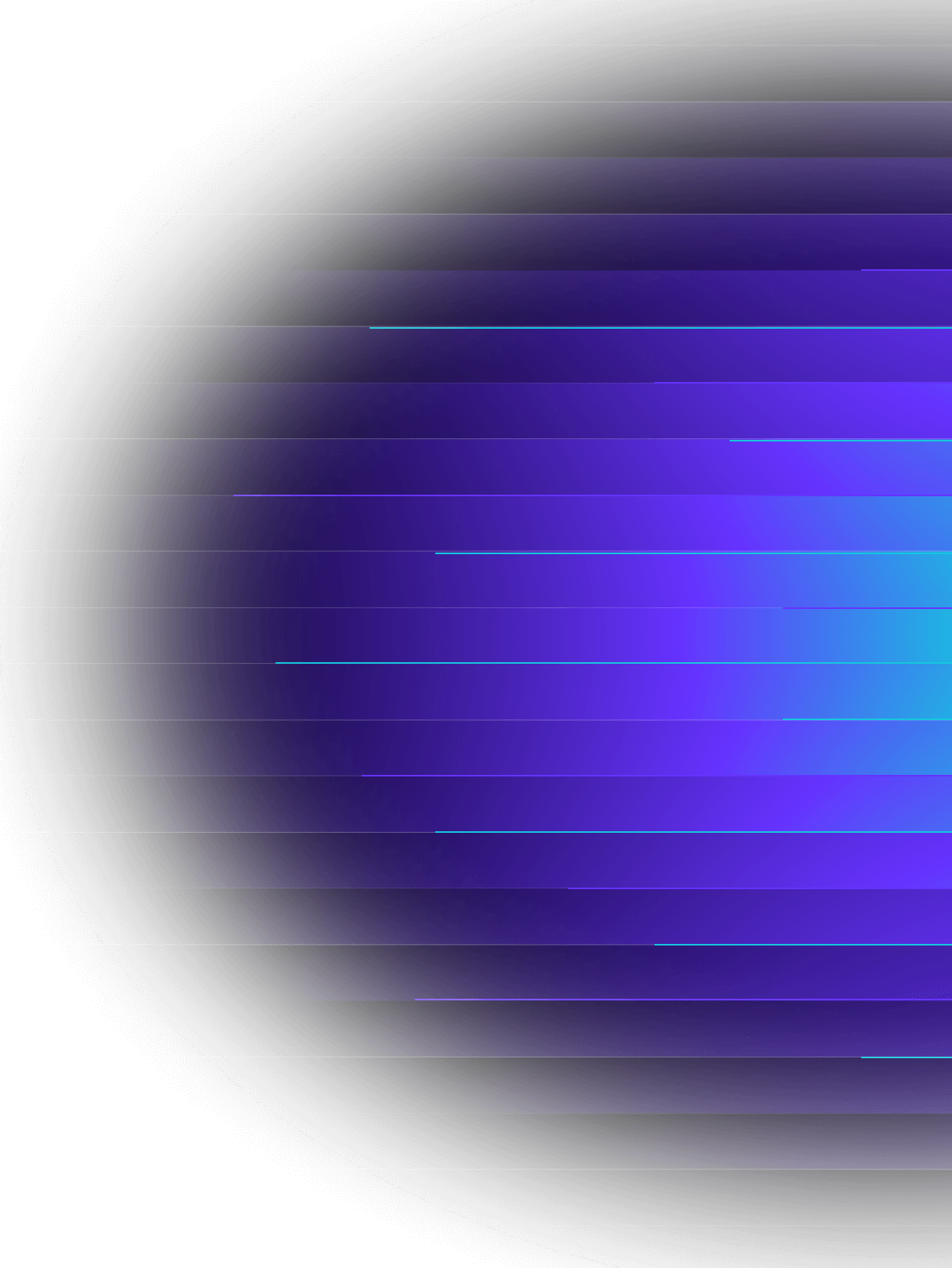
Using optical approaches to measure brain activity is a powerful technique, but it requires the subject’s head to be fixed under a microscope and for the skull to remain open, making it impractical for reading signals in realistic situations. Being able to predict the same information based solely on surface electrical readings would greatly expand the practicality.
“Our technology makes it possible to conduct longer duration experiments in which the subject is free to move around and perform complex behavioral tasks,” said Mehrdad Ramezani, co-first author of a paper in Nature Nanotechnology on the research. “This can provide a more comprehensive understanding of neural activity in dynamic, real-world scenarios.”
The technology is still a long way from use in humans though. At present, the team has only demonstrated the ability to learn correlations between optical and electrical signals recorded in individual mice. It’s unlikely this model could be used to predict deep brain activity from surface signals in a different mouse, let alone a person.
That means all individuals would have to undergo the fairly invasive data collection process before the approach would work. The authors admit more needs to be done to find higher level connections between the optical and electrical data that would allow models to generalize across individuals.
But given rapid improvements in the technology required to carry out both optical and electrical readings from the brain, it might not be long until the approach becomes more feasible. And it could ultimately strike a better balance between fidelity and invasiveness than competing technologies.
Image Credit: A thin, transparent, flexible brain implant sits on the surface of the brain to avoid damaging it, but with the help of AI, it can still infer activity deep below the surface. David Baillot/UC San Diego Jacobs School of Engineering
What we’re reading