Forget humans versus machines: humans plus machines is what will drive society forward. This was the central message conveyed by Dr. John Kelly, senior vice president of IBM Research, at the Augmenting Human Intelligence Cognitive Colloquium, which took place yesterday in San Francisco.
Organized by IBM, the colloquium brought together machine learning leaders in industry and academia to discuss how artificial intelligence can augment human intelligence — by helping us make sense of the quintillion bytes of data generated each day.

It’s not about machines gaining intelligence or taking over the world, said Kelly. It’s not even about recreating the human brain or its basic architecture. It’s about taking inspiration from the brain — or whatever inspiration from wherever we can get it — and changing the current computing architecture to better handle data and further our understanding of the world.
“I think the key question is: What’s the price of not knowing?” asked Kelly.
Around 80% of data is unstructured, meaning that current computing systems can’t make sense of it. By 2020, this number will reach 93%. To a human, unstructured data is far from enigmatic — think of describing a video recording of a street scene to a friend. Easy. To a current computer, however, the task is nearly insurmountable.
Yet analyzing unstructured data is far from a theoretical problem.
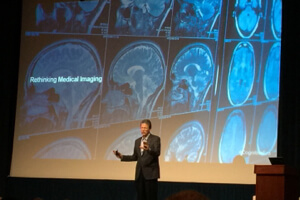
Take medicine, for example. In a single lifetime, a person can generate over one million gigabytes of health-related data, mostly in the form of electronic records and medical images. Multiply this by our current population, and the “secret to well being” may be hidden among this data, says Kelly. Yet, we don’t have the means to analyze, interpret and extrapolate from this vast resource.
The problem lies in both hardware and software. The challenge is formidable, said Dr. Yoshua Bengio, a neural network and deep learning expert at the University of Montreal and invited speaker. But scientists are making headway on both fronts.
A Brainy Chip
Currently, the basic unit of computer computation — the silicon chip — relies on the same outdated computational architecture that was first proposed nearly 70 years ago. These chips separate processing and memory — the two main functions that chips carry out — into different physical regions, which necessitates constant communications between the regions and lowers efficiency. Although this organization is sufficient for basic number crunching and tackling spreadsheets, it falters when fed torrents of unstructured data, as in vision and language processing.
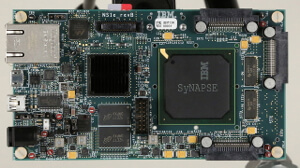
This is why we took the long and winding road to a production-scale neuromorphic computing chip, said Dr. Dharmendra Modha, chief scientist at IBM. Published last year in the prestigious journal Science, Modha and colleagues at IBM and Cornell University described a chip, TrueNorth, which works more like a mammalian brain than the tiny electronic chips that currently inhabit our smartphones.
When you look at the brain, it’s both digital and analog, said Dr. Terry Sejnowski, a pioneer in computational neuroscience at the Salk Institute and invited speaker.
It’s digital in the sense that it processes electrical spikes, especially for information that needs to travel long distances without decay. But it’s also analog in how it integrates information. It’s quite noisy, can be very imprecise, but it gets by really well by producing “ok” solutions under strict energy constraints — something that completely evades current computer chips
The brain is also a master at parallel computing and capable of dealing with immense complexity. Part of this is due to how neurons — the brain’s basic computational units — are dynamically connected. Each individual neuron talks to thousands of neighboring ones through chemical signals at synapses. A message can ripple through the brain’s 100 billion neurons and 100 trillion of synapses without the need for pre-programming: neuronal networks that fire together regularly are reinforced, whereas those that don’t are trimmed away.
It’s a highly efficient, adaptable and energy efficient computing architecture distributed across multiple processing levels and physical regions of the brain.
This means that there’s less need to shuttle data from one region to another, said Sejnowski.
TrueNorth mimics the brain by wiring 5.4 billion transistors into 1 million “neurons” that connect to each other via 256 million “synapses.” The chip doesn’t yet have the ability to incorporate dynamic changes in synaptic strength, but the team is working towards it.
“The chip is a fundamental departure from current architectures,” says Modha. But he stresses that it’s not a precise interpretation of the brain.
It’s sexy to think that we can go from AI to biology, but TrueNorth doesn’t model the brain. The brain is very good at some things — image perception, intuition, reasoning, even a sense of morality — but inept at making sense of vast amounts of data.
We’re trying to augment human intelligence with AI, not replicate it, stressed Modha.
Intuition-driven software
Scientists are also taking inspiration from the brain to work towards smarter algorithms.

There’re a lot of hard problems in AI, like generalizing from what’s been learned and reasoning out logical problems in “natural,” everyday language, says Bengio. Real-time online learning, at the speed of human decision making (roughly 50ms), is another tough nut to crack, as is efficient multi-module processing — that is, linking visual data with audio streams and other kinds of sensors.
Yet the machine learning panel was reluctant to identify fundamental limitations of deep learning. “Until there’s mathematical proof, I can’t say what’s impossible with the strategy,” laughed Bengio.
The field is steadily pushing forward. We’re now integrating memory storage into our recurrent networks to better deal with language translation and other problems that were intractable just a few years ago, says Bengio.
An important next question is to understand “why,” that is, how the algorithms are building representations to produce their answers.
The thing is, people want to know why a computer makes one decision or another before they trust them. Commuters, for example, want to know why a driverless car suddenly stops in the middle of the road, or ventures onto an unusual route. We fear what we don’t know, and that’s a problem for adopting new technology, agrees the panel.
Yet currently algorithm-generated representations are very difficult for humans to grasp, and their train of reasoning is hidden behind millions of computations. I think progress in natural language processing will help with this, says Bengio, in that computers can talk back to us.
The “black box” nature of deep learning algorithms also imposes a “magical” creative quality to the field. We’re all experimentalists, conceded the experts. The field mostly moves forward via human intuition; if it works, the scientists turn around and try to figure out the underlying theory.
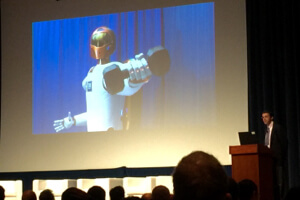
It’s a great example of human and machine synergy. Human intuition drives machines forward, and machines in turn augment human intelligence with interpretable data.
We’re building sophisticated, autonomous and intelligent systems that are extensions and collaborators of ourselves, says Dr. Myron Diftler, a scientist that constructs robots at the NASA Johnson Space Center in a panel discussion.
It’s a humans plus machines future.
Image Credit: Shutterstock.com; IBM/Flickr; Shelly Fan