Nvidia’s Fake Faces Are a Masterpiece—But Have Deeper Implications

‘Don’t believe everything you see on the internet’ is pretty standard advice, but it’s getting harder than ever to distinguish the real from the fake. A new algorithm from Nvidia could muddy the waters further by generating completely made-up human faces that are almost indistinguishable from the real thing.
AI’s ability to synthesize, swap, and morph images, video, and even speech has come on in leaps and bounds in recent years. That’s driving more powerful image editing software, more realistic voice assistants, and even opening the door to automatically generating entire digital worlds. But there’s also growing concern that as these kinds of tools become increasingly sophisticated and accessible, they’re eroding our ability to trust these mediums.
The problem was thrust into the public consciousness by the Deepfake scandal that broke this time last year, when AI was used to superimpose celebrities’ faces onto porn videos. Since then, similar techniques have been used to put words into the mouths of politicians, and there has been widespread hand-wringing about the technology’s potential impact in an era of fake news and digital manipulation.
GANs
The class of algorithms at the heart of the most advanced of these solutions are called generative adversarial networks, or GANs, and they also form the core of the new Nvidia software. Essentially, GANs pit two neural networks against each other, one designed to spot synthesized images and the other designed to create fakes realistic enough to slip past undetected. This game of cat and mouse is repeated over and over again, with the synthesized images getting steadily more realistic.
The approach was only invented in 2014, but there’s been rapid progress since then, from grainy black and white passport photos to hi-res, full-color (if sometimes slightly wonky) head shots. But the latest advance from Nvidia has achieved an unprecedented level of realism—you’d be hard-pressed to tell the output apart from images pulled out of a stock photo catalog.
The researchers’ main innovation was to combine their GAN with methods from the field of style transfer—something you may be familiar with from apps that rework your photos into the style of Vincent van Gogh or some other artist. These approaches let neural networks learn to separate the content and the style of an image and then recombine them in interesting ways.
Leveling Up
Most neural networks designed to work with images “understand” them in terms of a hierarchy of features, starting with broad strokes like their pose, then things like the distance between their eyes and nose, and at the lowest level details like the tone of their skin. By adding the style transfer methods the new algorithm is essentially able to learn styles for each of these feature levels.
Researchers can then remix these various styles at different levels to create entirely new faces, or simply change the color of someone’s hair by tweaking the low-level style. The researchers also tested the approach on other image datasets, creating convincing forgeries of cars and bedrooms as well.
But as cool as conjuring new faces out of thin air is, that’s not the real motivation for the research. Despite the improvements in the technology, GANs—and neural networks more broadly—still operate as black boxes insofar as we don’t really understand what it is they’re focusing on in an image.
As Tiernan Ray notes in ZDNet, by forcing their network to separate what it is focusing on into high- and low-level features and then making it possible to swap them around, we’re able to get a much better sense of what the algorithm is looking at at each level of abstraction.
But while that might be of great interest to computer scientists, what’s likely to be a more pressing concern for the rest of us is what the practical side-effects of the approach are, something the authors conspicuously avoid discussing in their paper.
Be Part of the Future
Sign up to receive top stories about groundbreaking technologies and visionary thinkers from SingularityHub.
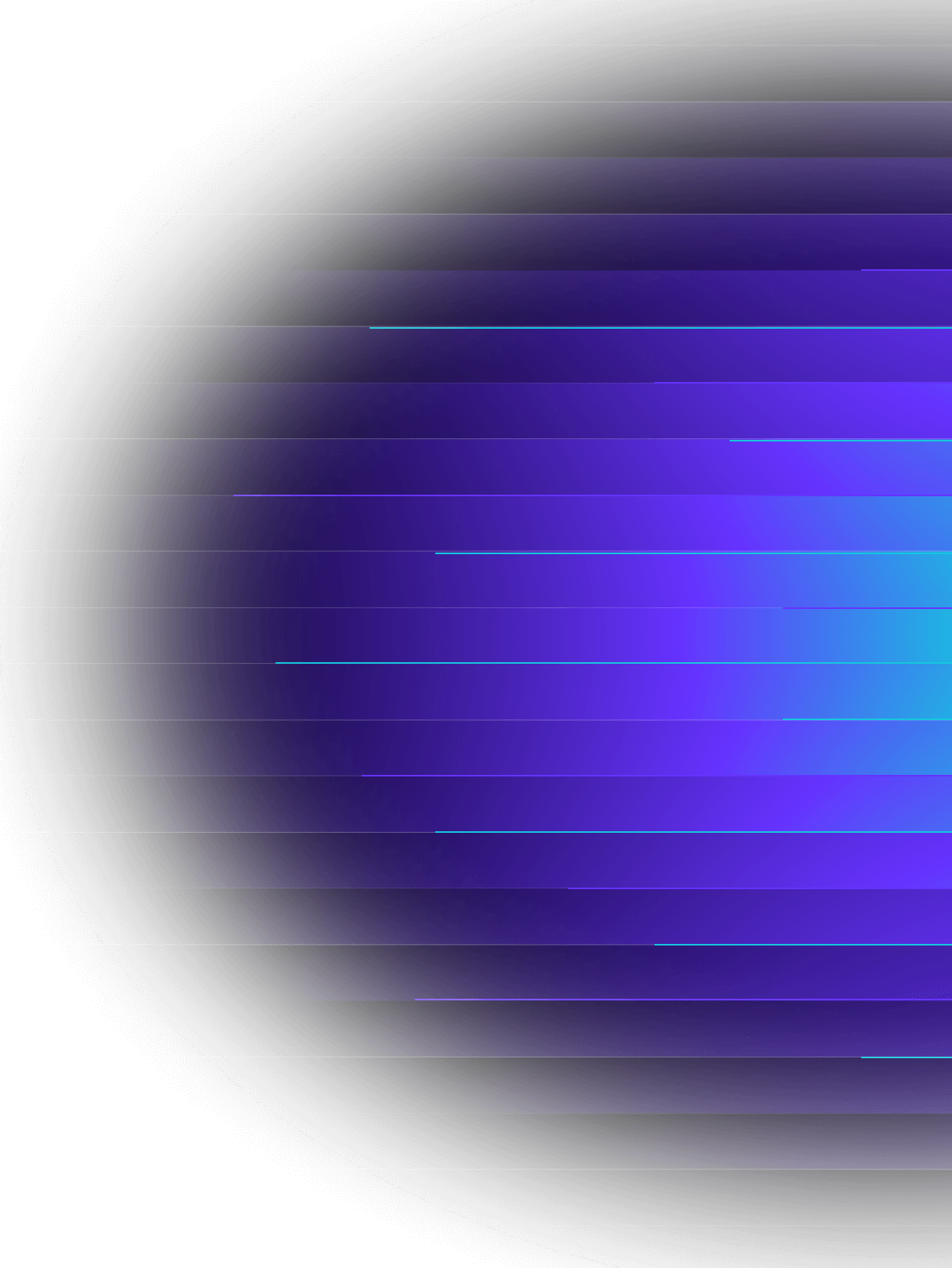
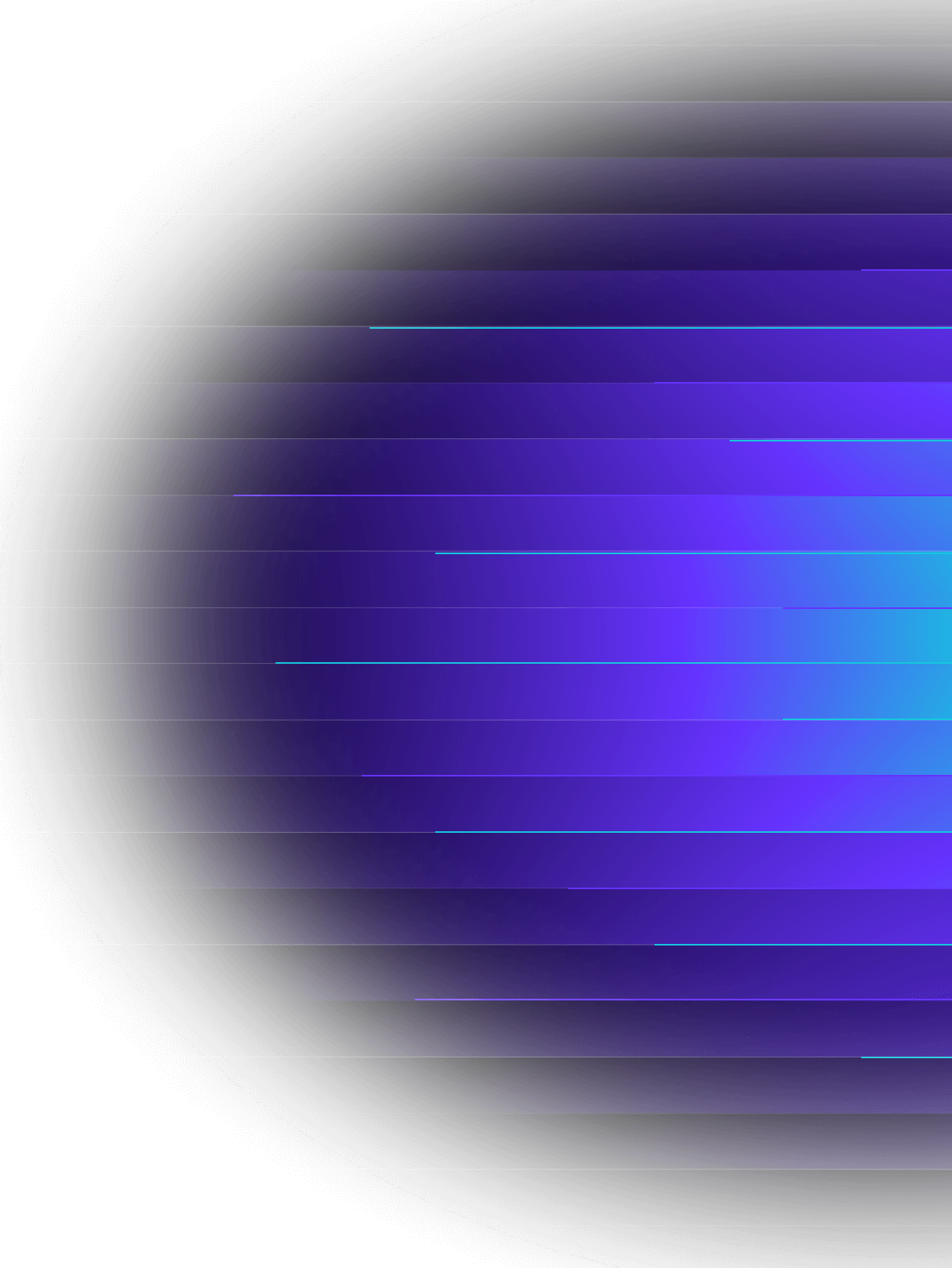
Admittedly the fake faces aren’t perfect yet—a blog post from artist-coder Kyle McDonald notes subtle aberrations, like phantom earrings and weird teeth that become clear on closer inspection—but they are good enough that they would fool most people most of the time.
Why It Matters
One potential application of that kind of trickery noted by The Register could be to create highly realistic profile photos for fake social media accounts used to manipulate online discourse. And with the authors planning to release the source code, that ability could soon be freely available.
Unlike the Deepfakes code, though, which allowed anyone with a relatively powerful graphics card to start creating their own videos, training this new model took nearly a week on eight of Nvidia’s cutting-edge Tesla GPU chips. That means the costs will likely outweigh the benefits for most conceivable applications.
But the promise and the danger of this approach is probably not so much in it’s direct application, but the discovery that incorporating style transfer methods can lead to GANs capable of much higher-fidelity output. That’s likely to inspire the development of a new generation of image and video-spoofing algorithms whose outputs are even harder to detect.
To rework a famous saying, a fake picture is worth a thousand fake words, and with the increasing democratization of this kind of technology its going to become harder and harder to trust what we see on the web. As Joshua Rothman notes in the New Yorker, that presents a double-edge sword—not only will people be able to create forgeries to twist the public discourse, public figures will also have plausible deniability for anything they’re caught doing on camera.
‘Don’t believe everything you see on the internet’ could soon shift to ‘don’t believe anything you see on the internet.’
Image Credit: ESB Professional / Shutterstock.com
What we’re reading