Language and speech are how we express our inner thoughts. But neuroscientists just bypassed the need for audible speech, at least in the lab. Instead, they directly tapped into the biological machine that generates language and ideas: the brain.
Using brain scans and a hefty dose of machine learning, a team from the University of Texas at Austin developed a “language decoder” that captures the gist of what a person hears based on their brain activation patterns alone. Far from a one-trick pony, the decoder can also translate imagined speech, and even generate descriptive subtitles for silent movies using neural activity.
Here’s the kicker: the method doesn’t require surgery. Rather than relying on implanted electrodes, which listen in on electrical bursts directly from neurons, the neurotechnology uses functional magnetic resonance imaging (fMRI), a completely non-invasive procedure, to generate brain maps that correspond to language.
To be clear, the technology isn’t mind reading. In each case, the decoder produces paraphrases that capture the general idea of a sentence or paragraph. It does not reproduce every single word. Yet that’s also the decoder’s power.
“We think that the decoder represents something deeper than languages,” said lead study author Dr. Alexander Huth in a press briefing. “We can recover the overall idea…and see how the idea evolves, even if the exact words get lost.”
The study, published this week in Nature Neuroscience, represents a powerful first push into non-invasive brain-machine interfaces for decoding language—a notoriously difficult problem. With further development, the technology could help those who have lost the ability to speak to regain their ability to communicate with the outside world.
The work also opens new avenues for learning about how language is encoded in the brain, and for AI scientists to dig into the “black box” of machine learning models that process speech and language.
“It was a long time coming…we were kinda shocked that this worked as well as it does,” said Huth.
Decoding Language
Translating brain activity to speech isn’t new. One previous study used electrodes placed directly in the brains of patients with paralysis. By listening in on the neurons’ electrical chattering, the team was able to reconstruct full words from the patient.
Huth decided to take an alternative, if daring, route. Instead of relying on neurosurgery, he opted for a non-invasive approach: fMRI.
“The expectation among neuroscientists in general that you can do this kind of thing with fMRI is pretty low,” said Huth.
There are plenty of reasons. Unlike implants that tap directly into neural activity, fMRI measures how oxygen levels in the blood change. This is called the BOLD signal. Because more active brain regions require more oxygen, BOLD responses act as a reliable proxy for neural activity. But it comes with problems. The signals are sluggish compared to measuring electrical bursts, and the signals can be noisy.
Yet fMRI has a massive perk compared to brain implants: it can monitor the entire brain at high resolution. Compared to gathering data from a nugget in one region, it provides a birds-eye view of higher-level cognitive functions—including language.
With decoding language, most previous studies tapped into the motor cortex, an area that controls how the mouth and larynx move to generate speech, or more “surface level” in language processing for articulation. Huth’s team decided to go one abstraction up: into the realm of thoughts and ideas.
Into the Unknown
The team realized they needed two things from the onset. One, a dataset of high-quality brain scans to train the decoder. Two, a machine learning framework to process the data.
To generate the brain map database, seven volunteers had their brains repeatedly scanned as they listened to podcast stories while having their neural activity measured inside an MRI machine. Laying inside a giant, noisy magnet isn’t fun for anyone, and the team took care to keep the volunteers interested and alert, since attention factors into decoding.
For each person, the ensuing massive dataset was fed into a framework powered by machine learning. Thanks to the recent explosion in machine learning models that help process natural language, the team was able to harness those resources and readily build the decoder.
It’s got multiple components. The first is an encoding model using the original GPT, the predecessor to the massively popular ChatGPT. The model takes each word and predicts how the brain will respond. Here, the team fine-tuned GPT using over 200 million total words from Reddit comments and podcasts.
This second part uses a popular technique in machine learning called Bayesian decoding. The algorithm guesses the next word based on a previous sequence and uses the guessed word to check the brain’s actual response.
For example, one podcast episode had “my dad doesn’t need it…” as a storyline. When fed into the decoder as a prompt, it came with potential responses: “much,” “right,” “since,” and so on. Comparing predicted brain activity with each word to that generated from the actual word helped the decoder hone in on each person’s brain activity patterns and correct for mistakes.
After repeating the process with the best predicted words, the decoding aspect of the program eventually learned each person’s unique “neural fingerprint” for how they process language.
A Neuro Translator
As a proof of concept, the team pitted the decoded responses against the actual story text.
It came surprisingly close, but only for the general gist. For example, one story line, “we start to trade stories about our lives we’re both from up north,” was decoded as “we started talking about our experiences in the area he was born in I was from the north.”
This paraphrasing is expected, explained Huth. Because fMRI is rather noisy and sluggish, it’s nearly impossible to capture and decode each word. The decoder is fed a mishmash of words and needs to disentangle their meanings using features like turns of phrase.
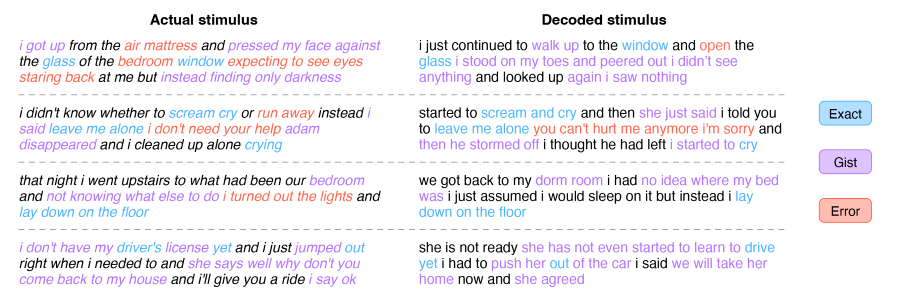
In contrast, ideas are more permanent and change relatively slowly. Because fMRI has a lag when measuring neural activity, it captures abstract concepts and thoughts better than specific words.
This high-level approach has perks. While lacking fidelity, the decoder captures a higher level of language representation than previous attempts, including for tasks not limited to speech alone. In one test, the volunteers watched an animated clip of a girl being attacked by dragons without any sound. Using brain activity alone, the decoder described the scene from the protagonist’s perspective as a text-based story. In other words, the decoder was able to translate visual information directly into a narrative based on a representation of language encoded in brain activity.
Similarly, the decoder also reconstructed one-minute-long imagined stories from the volunteers.
After over a decade working on the technology, “it was shocking and exciting when it finally did work,” said Huth.
Although the decoder doesn’t exactly read minds, the team was careful to assess mental privacy. In a series of tests, they found that the decoder only worked with the volunteers’ active mental participation. Asking participants to count up by an order of seven, name different animals, or mentally construct their own stories rapidly degraded the decoder, said first author Jerry Tang. In other words, the decoder can be “consciously resisted.”
For now, the technology only works after months of careful brain scans in a loudly humming machine while lying completely still—hardly feasible for clinical use. The team is working on translating the technology to fNIRS (functional near-infrared spectroscopy), which measures blood oxygen levels in the brain. Although it has a lower resolution than fMRI, fNIRS is far more portable as the main hardware is a swimming-cap-like device that easily fits under a hoodie.
“With tweaks, we should be able to translate the current setup to fNIRS wholesale,” said Huth.
The team is also planning to use newer language models to boost the decoder’s accuracy, and potentially bridge different languages. Because languages have a shared neural representation in the brain, the decoder could in theory encode one language and use the neural signals to decode it into another.
It’s an “exciting future direction,” said Huth.
Image Credit: Jerry Tang/Martha Morales/The University of Texas at Austin