This AI-Powered Exoskeleton Could Speed Adoption by the Masses

Exoskeletons could help disabled people move freely again and one day boost the power and stamina of workers doing manual labor. A new AI-powered approach to building these devices could help expand their use.
While the term exoskeleton might evoke images from sci-fi movies of people ensconced in massive robotic bodies, the real-world version tends to be more modest. Typically, these devices feature a few robotic hinges strapped to the wearer’s waist, where they add power to a person's legs while walking, running, or climbing stairs.
But ensuring these devices provide extra juice at the right time is trickier than it looks and requires a detailed understanding of the wearer’s biomechanics. This is often gained by training machine learning algorithms on data collected from humans while wearing the device, but it’s time-consuming and costly to gather.
A new “experiment-free” approach does away with the need for this data and trains the AI model in simulation instead. This should dramatically shorten the development cycle for the technology, say the authors of a new paper on the technique in Nature.
“Exoskeletons have enormous potential to improve human locomotive performance,” North Carolina State University’s Hao Su said in a press release.
“However, their development and broad dissemination are limited by the requirement for lengthy human tests and handcrafted control laws. The key idea here is that the embodied AI in a portable exoskeleton is learning how to help people walk, run, or climb in a computer simulation, without requiring any experiments.”
Historically, the software that controls exoskeletons has had to be carefully programmed for specific activities and painstakingly calibrated to individual users. This typically takes hours of human testing in specialized laboratories, which significantly slows down both research and deployment.
Recently, researchers showed they could create an AI-powered universal controller that can seamlessly adapt to new users without extra training. But it still required them to collect extensive data from 25 subjects to train the controller.
The new approach does away with the need for human input by instead training the controller in simulation. The set-up is fairly complex, involving neural networks trained on human movement data collected using cheap wearable sensors, a full-body musculoskeletal model, a physical model of the exoskeleton, and a model that simulates contact between the wearer and the exoskeleton.
Be Part of the Future
Sign up to receive top stories about groundbreaking technologies and visionary thinkers from SingularityHub.
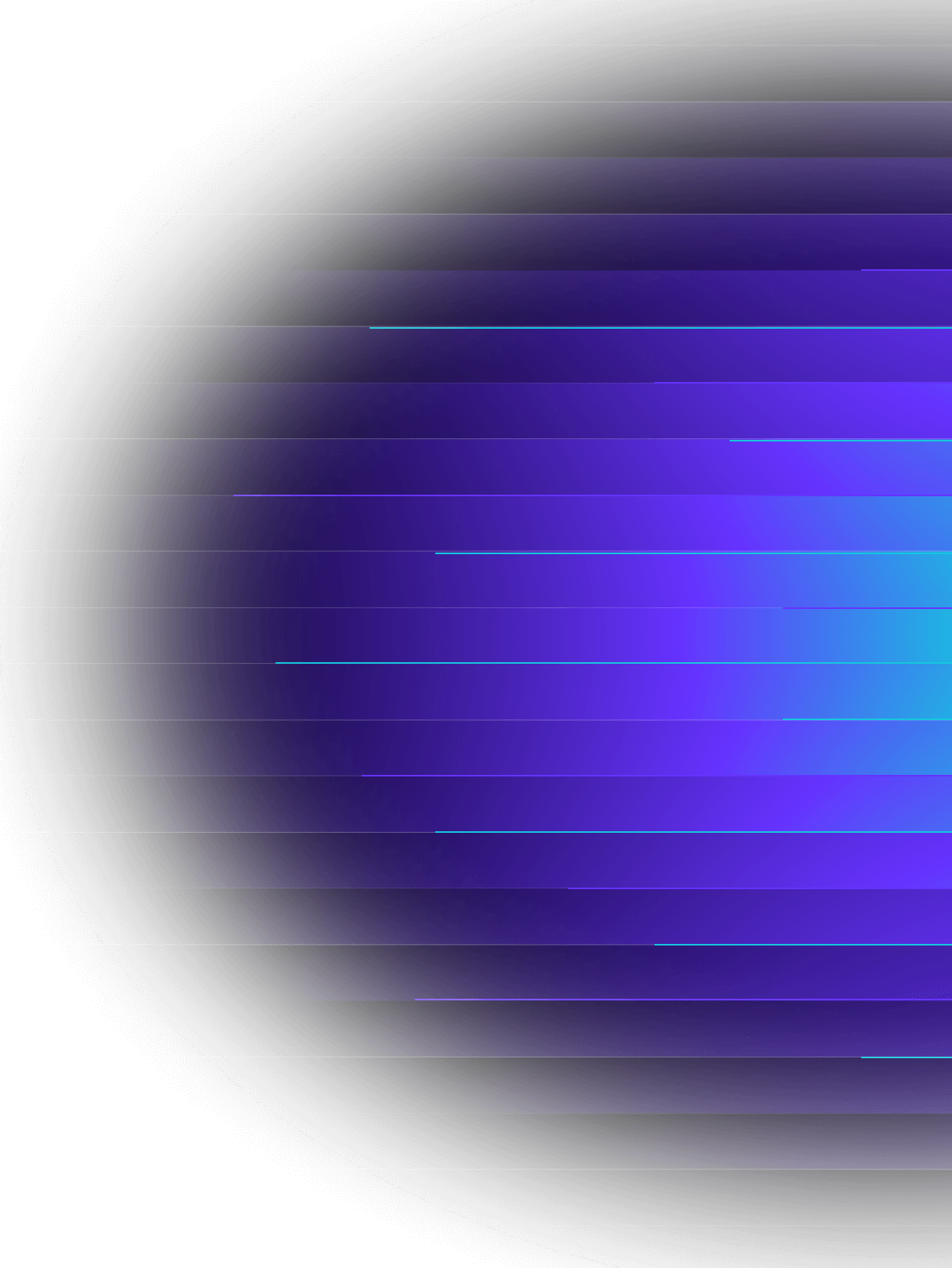
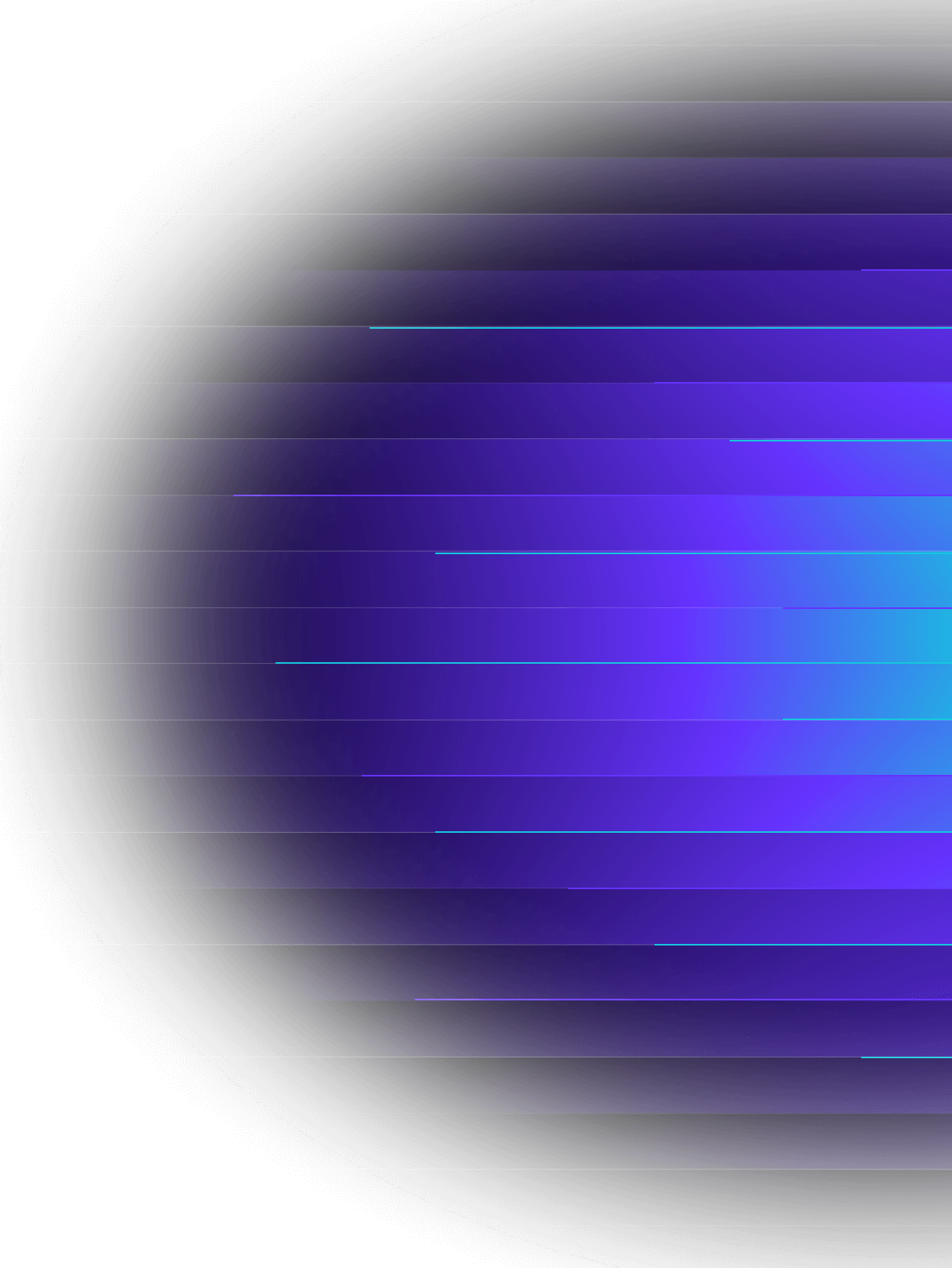
These are used to simulate a person wearing the exoskeleton walking, running, and climbing stairs. Over millions of virtual trials, reinforcement learning—a machine learning method, wherein an algorithm is rewarded for making progress toward a specified goal—trains a controller to exert the right amount of power at the right time to boost the efficiency of the wearer. The entire process takes just eight hours on a single GPU.
The resulting model is user agnostic, automatically adapting to the unique movements patterns of different people. And it can transition seamlessly between the three activities, unlike previous approaches where the user has had to manually set it to different modes.
In tests, the team showed that people used 24 percent less energy when walking using the robotic exoskeleton compared to when they walked unaided. They also used 13 percent less energy when running and 15 percent less when climbing stairs.
Training AI in simulations for work in the real world is notoriously difficult, so a significant performance boost is a huge achievement. And the team says their approach should readily translate to other kinds of activities and different exoskeletons.
For now, the researchers are focused on improving exoskeletons for older adults and people with neurological conditions. But it’s not hard to see the broader applications of a technology that can dramatically increase the power and efficiency of human movement.
Image Credit: Hao Su / NC State University
What we’re reading