How the Crowd Taught a Robot to Build a LEGO Turtle

Humans learn by imitation. There’s no predicting what your kid will bring home from school or the park. What if machines could learn like kids? In fact, they can and do. Robots don’t go to the park or school (yet)—but they live in labs and can go online.
University of Washington researchers recently augmented typical in-person imitation learning (where a human shows a robot how to solve a problem) with online crowdsourcing to teach their robots how to build shapes with blocks.
In a paper describing their work, the group says in-person imitation learning together with crowdsourced learning realized better results than in-person imitation alone.
After the robot was taught by 14 volunteers in the lab, the scientists posted questions to Amazon Mechanical Turk. Mechanical Turk distributes simple tasks to thousands of online workers for a small fee per completed task.
The researchers asked users, “How would you make a shape (turtle, person, car, etc.) using these colored blocks?” Their software analyzed hundreds of responses and sorted the best designs by asking participants to rate designs submitted by other users. The program chose the most highly rated responses and built them using physical blocks.
Crowdsourcing learning for robots could be a powerful technique. The current process involves fewer teachers and can be expensive. But for now, crowdsourcing is likely best combined with other methods and at least some human supervision.
Be Part of the Future
Sign up to receive top stories about groundbreaking technologies and visionary thinkers from SingularityHub.
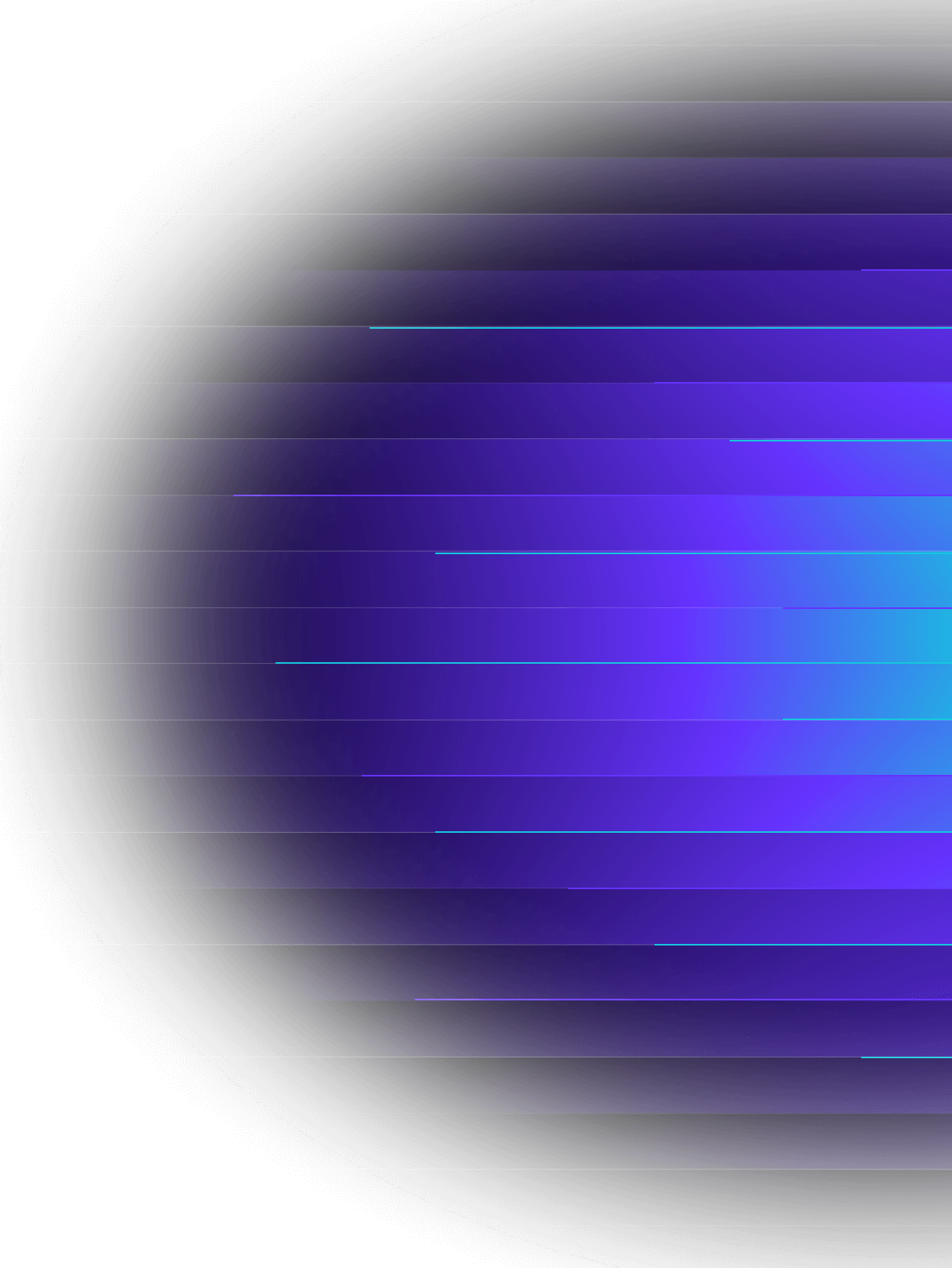
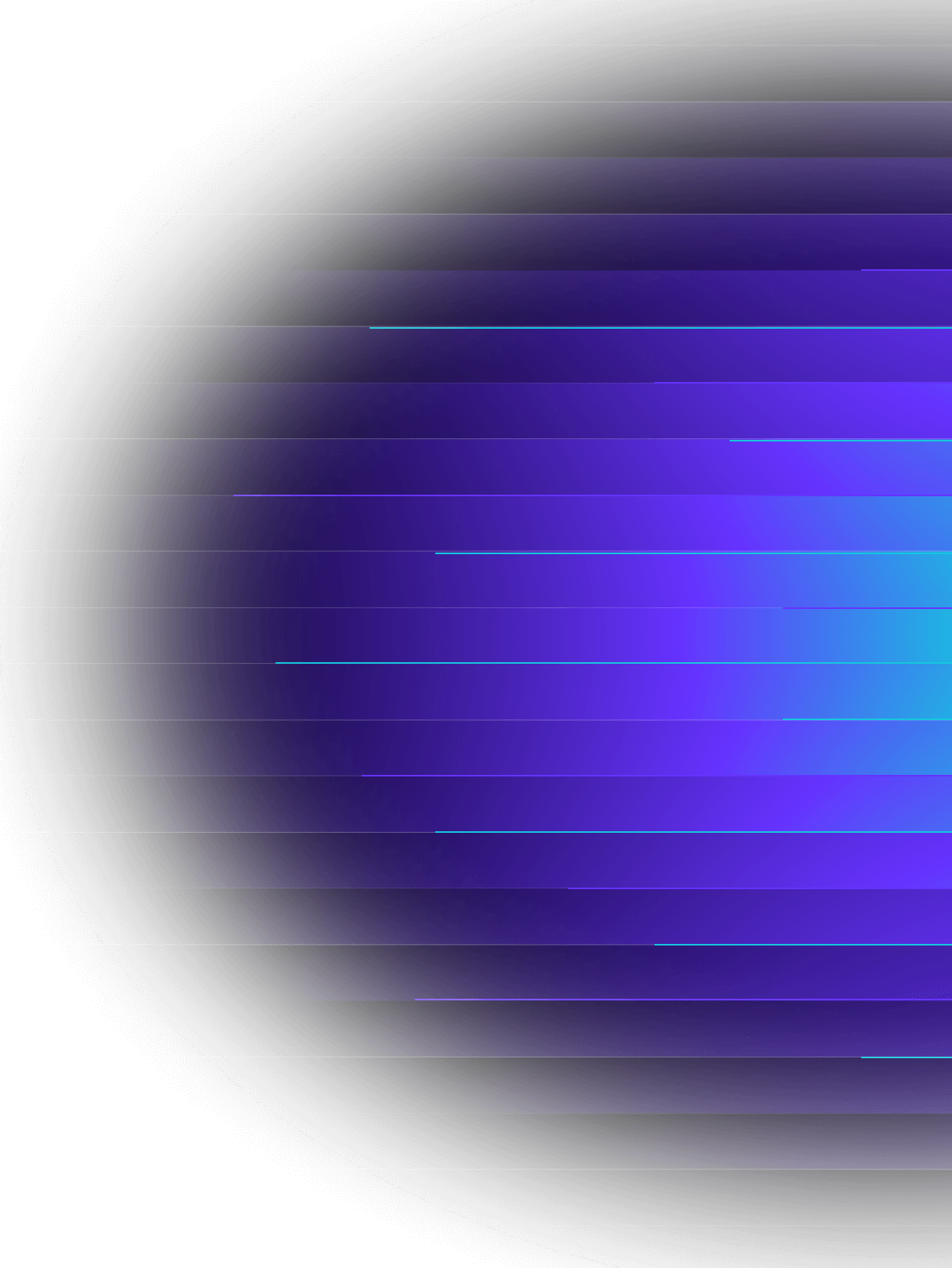
For example, at first, crowdsourced learning seems useful for projects like Google’s self-driving cars. Currently, Google engineers log miles on the road and laboriously catalogue and write code for as many situations as they can. They're able to anticipate a variety of events, but can’t possibly account for everything.
What if Google’s cars learned to solve rare, low-probability events from the crowd? As it turns out, what works for a robot playing with blocks might be impractical for a multi-ton machine trying to safely navigate city streets. Quality control is crucial.
The authors note in their paper, “Although we demonstrated the benefits of utilizing crowdsourcing, the context of robotic imitation learning, crowdsourcing needs to be used with caution. The quality control of crowdsourcing was non-trivial.”
That said, crowdsourced machine learning could help a robot better sort boxes in a warehouse. And maybe Google’s self-driving cars could use even more closely supervised crowdsourcing. The system queries the crowd, selects what it thinks are the best solutions, and engineers give the final thumbs up.
As robots become commonplace, however, we imagine they might cut humans out of the learning equation entirely—that is, as robots interact with us day to day, they analyze what works and what doesn't, adjust their programming, and share it with each other. Robots would get more capable the more they interact with the world.
Image Credit: "Accelerating Imitation Learning through Crowdsourcing"/University of Washington
Jason is editorial director at SingularityHub. He researched and wrote about finance and economics before moving on to science and technology. He's curious about pretty much everything, but especially loves learning about and sharing big ideas and advances in artificial intelligence, computing, robotics, biotech, neuroscience, and space.
What we’re reading