Thanks to deep learning, the tricky business of making brain atlases just got a lot easier.
Brain maps are all the rage these days. From rainbow-colored dots that highlight neurons or gene expression across the brain, to neon “brush strokes” that represent neural connections, every few months seem to welcome a new brain map.
Without doubt, these maps are invaluable for connecting the macro (the brain’s architecture) to the micro (genetic profiles, protein expression, neural networks) across space and time. Scientists can now compare brain images from their own experiments to a standard resource. This is a critical first step in, for example, developing algorithms that can spot brain tumors, or understanding how depression changes brain connectivity. We’re literally in a new age of neuro-exploration.
But dotting neurons and drawing circuits is just the start. To be truly useful, brain atlases need to be fully annotated. Just as early cartographers labeled the Earth’s continents, a first step in annotating brain maps is to precisely parse out different functional regions.
Unfortunately, microscopic neuroimages look nothing like the brain anatomy coloring books. Rather, they come in a wide variety of sizes, rotations, and colors. The imaged brain sections, due to extensive chemical pre-treatment, are often distorted or missing pieces. To ensure labeling accuracy, scientists often have to go in and hand-annotate every single image. Similar to the pain of manually labeling data for machine learning, this step creates a time-consuming, labor-intensive bottleneck in neuro-cartography endeavors.
No more. This month, a team from the Brain Research Institute of UZH in Zurich tapped the processing power of artificial brains to take over the much-hated job of “region segmentations.” The team fed a deep neural net microscope images of whole mouse brains, which were “stained” with a variety of methods and a large pool of different markers.
Regardless of age, method, or marker, the algorithm reliably identified dozens of regions across the brain, often matching the performance of human annotation. The bot also showed a remarkable ability to “transfer” its learning: trained on one marker, it could generalize to other markers or staining. When tested on a pool of human brain scans, the algorithm performed just as well.
“Our…method can accelerate brain-wide exploration of region-specific changes in brain development and, by easily segmenting brain regions of interest for high-throughput brain-wide analysis, offer an alternative to existing complex … techniques,” the authors said.
Um…So What?
To answer that question, we need to travel back to 2010, when the Allen Brain Institute released the first human brain map. A masterpiece 10 years in the making, the map “merged” images of six human brains into a single, annotated atlas that combined the brain’s architecture with dots representing each of the 10,000 genes across the brain.
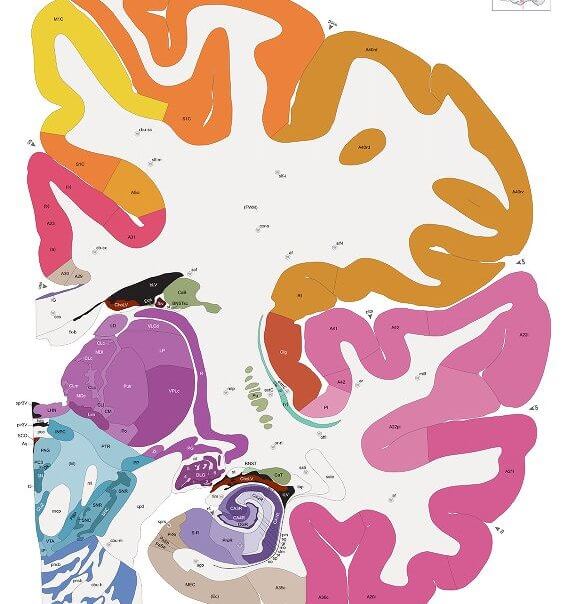
It was pretty to look at, sure. But back then, even the project architect Dr. Amy Bernard didn’t know how the atlas could be used outside of Wikipedia-esque browsing. After all, it’s hard to generalize all the nuances of each individual brain from just six.
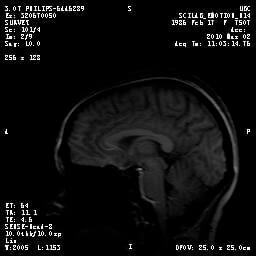
Then in late 2016, a team from Cambridge figured out how to “sync up” MRI scans from teenagers’ brains to Bernard’s genetic brain map. MRI looks at large-scale things; but now, scientists could also parse the genetic changes in the teenagers’ various brain regions by combining the two maps. In other words, if scientists have a way to match their own maps to reference atlases, such as those from the Allen Brain Institute, the results can be extrapolated to any individual, regardless of the particular quirks of each single brain.
The hard part is the “syncing up” step, which is also known as “image registration.” This is why the new study matters. For whatever problem they’re studying—a certain neurological disorder, a particular neural cell type, a gene linked to brain development—scientists can now generate brain atlases using their own data for a specific goal, such as comparing a malfunctioning brain region with another at the genetic, cellular, connection, and structural level. If further verified, deep learning could become a first step that will eventually allow neuroscientists to stitch together multiple efforts at mapmaking.
SeBRe
The team took obvious inspiration from deep learning’s recent success in object recognition. Starting from a type of neural net called Mask R-CNN, the team pre-trained the network on an open-source bank of images unrelated to the brain. They then fed the algorithm brain images from the Allen Brain Institute online public resource, as well as a set of open-sourced MRI images.
Each input brain image was then passed through a series of processing stages in which layers of the deep neural net propose, classify, and then segment brain regions by extracting features. The whole process is remarkably similar to current AI methods that recognize objects. But because the algorithm deals with microscope images, it had to tackle extra issues.
One is the “labeling” process. Unlike the objects around us, without literally coloring the brain, it looks like a greyish blob of fat with curious patterns splattered across some areas. A trained eye can roughly tease out main brain regions, but to obtain finer detail, scientists often treat the brain slices with chemicals. Like tie-dying a shirt, some stain it purple, others greyish-silver. Another trick is to use fluorescent probes, which are targeted to proteins lined outside brain cells. These guys are a tad more powerful: because each brain cell type has a slightly different protein profile, they can be used to “mark” particular cells—those that excite or dampen electrical processes, for example, or those that pump out dopamine, serotonin, and other brainy chemicals that “tune” the brain’s transmissions.
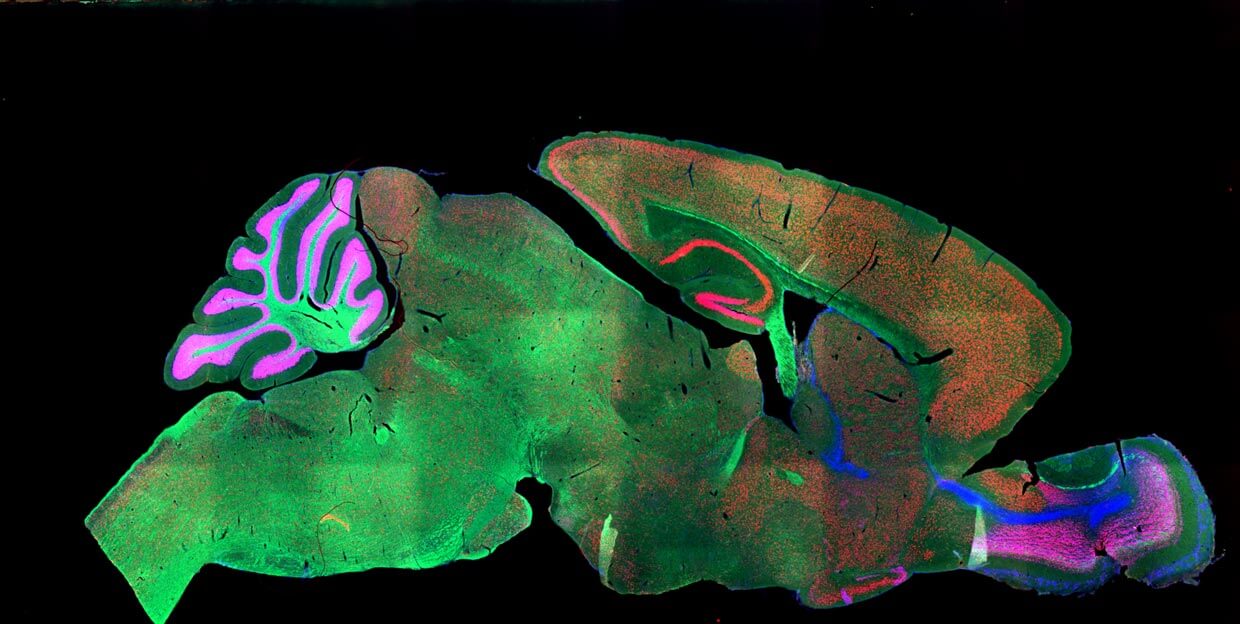
Still with me? Each lab often prefers a particular set of markers or dyes; this makes the final image drastically different. Because scientists often use a paint brush (literally) or other tool to stick a brain slice onto the glass slide, the specimens don’t always line up perfectly, and sometimes they’ll tear or crumple. Finally, since the brain is in 3D you can chop it up front-to-back or left-to-right, depending on what suits your needs. The result is a hodgepodge of images that look nothing like the reference atlas.
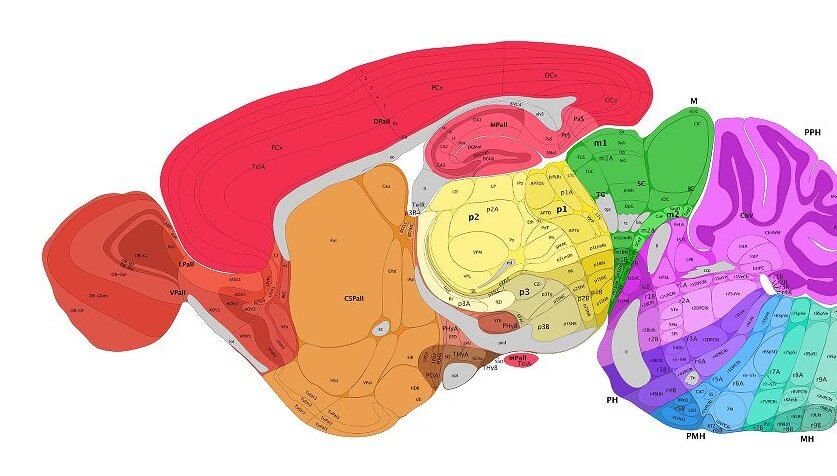
SeBRe solves the first two issues: regardless of what type of dye or fluorescent marker it’s trained on, the algorithm can tease out the cortex, hippocampus, basal ganglia (for movement) and other regions with roughly 85 percent precision.
It also showed a respectable ability to transfer its learning from one marker to another: when trained to segment brain regions by looking at inhibitory neurons, the algorithm performed equally well using excitatory markers, which generally have a slightly different distribution. SeBRe familiar with tie-dyed brains could also parse fluorescence-labeled brains. Finally, when trained solely on the brains of 14-day-old mice, the algorithm could also segment brain images from a myriad of other ages—something that takes humans significant practice to do.
Deep Learning Meets Brain Atlas
The algorithm, though impressive, didn’t perform well on all brain regions. Like an amateur neuroanatomist, it had trouble teasing apart landscapes in more “squished” brain parts packed with regional structures.
Even so, it’s a promising look at the future of compiling brain atlases. The algorithm can be used to pre-process images with different scales, “stretching” or “shrinking” individual pictures so that together they form a legible brain, a critical step in mapping brains during development or brains with shrinkage or deformities. The algorithm could even parse out substructures inside the hippocampus, something that takes substantial training to do across images.
Much like Google Maps, scientists may now have a way to “sync up” their experimental maps with other available resources. And similar to how Google Maps integrates a classic, terrain, street, and satellite view, brain map syncing will undoubtedly paint a much richer landscape of our brain’s landscape and connection highways, in health and disease.
Image Credit: A section of the mouse brain stained with fluorescent markers. NICHD/I. Williams / CC BY 2.0